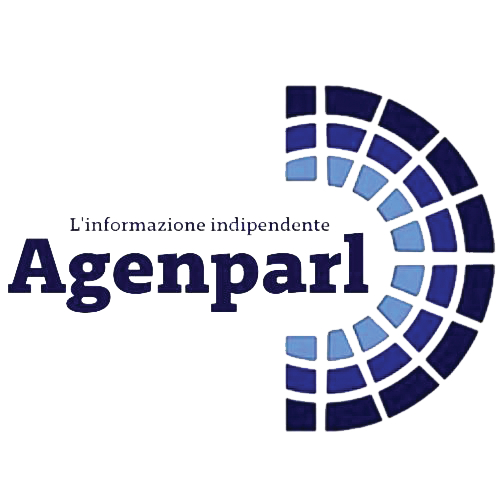
(AGENPARL) – gio 16 marzo 2023 Si invia in allegato “Banks’ liquidity transformation rate: determinants and impact on lending”, il trentaduesimo numero della collana “Mercati, infrastrutture, sistemi di pagamento”, di cui riportiamo in calce l’abstract.
La pubblicazione, in lingua inglese, è disponibile anche a questo [link](https://www.bancaditalia.it/media/notizia/il-tasso-di-trasformazione-della-liquidit-delle-banche-determinanti-e-effetti-sull-erogazione-del-credito/).
Cordiali saluti,
Divisione Relazioni con i media
Servizio Comunicazione
Banca d’Italia
Please find attached “Banks’ liquidity transformation rate: determinants and impact on lending”, the 32nd issue of the series “Markets, Infrastructures, Payment Systems”.
The paper, in English, is also available at this [link](https://www.bancaditalia.it/media/notizia/banks-liquidity-transformation-rate-determinants-and-impact-on-lending/?com.dotmarketing.htmlpage.language=1).
Abstract below.
Best regards,
Media relations Division
Communications Directorate
Banca d’Italia
Banks’ liquidity transformation rate: determinants and impact on lending
Numero 32 – marzo 2023
Issue 32 – March 2023
Sintesi
Il lavoro esamina l’interazione tra il liquidity coverage ratio (LCR), la composizione del collateral pool, le caratteristiche delle banche e l’attività di prestito. Il contributo è duplice. In primo luogo, si analizza come le caratteristiche delle banche siano correlate al loro tasso di trasformazione della liquidità. Il risultato principale è che le variabili relative alla liquidità e all’efficienza delle banche hanno un impatto sul loro tasso di trasformazione della liquidità, mentre non risulta alcun legame con gli indicatori di solidità patrimoniale. L’analisi conferma, pertanto, l’idea che le banche centrali possano fornire liquidità e ampliare la gamma di garanzie idonee, senza assumere rischi eccessivi nei loro bilanci. In secondo luogo, si esamina la correlazione tra il tasso di trasformazione della liquidità delle banche e l’offerta di credito. Dall’indagine emerge che le banche che impegnano più attività illiquide in cambio di riserve di banca centrale sono quelle che hanno aumentato maggiormente i loro prestiti all’economia, suggerendo che l’ampia gamma di garanzie idonee nell’area dell’euro avrebbe supportato l’erogazione di credito all’economia reale negli ultimi anni e, in particolare, durante la pandemia.
Abstract
This paper examines the interaction between the liquidity coverage ratio (LCR), the composition of the collateral pool, banks’ characteristics, and lending activity. Our contribution is twofold. Firstly, we analyse how banks’ characteristics correlate with their liquidity transformation rate. The main finding is that variables relating to banks’ liquidity and efficiency have an impact on their liquidity transformation rate, though we did not find any link with financial soundness indicators. This supports the view that central banks can provide liquidity and relax the collateral framework without taking on excessive risks on their balance sheets. Secondly, we investigate how banks’ liquidity transformation rate correlates to credit supply. Our findings show that the banks that pledged more illiquid assets against central bank reserves are those that increased their lending to the economy the most, suggesting that the broad range of eligible collateral in the euro area may have supported the provision of credit to the real economy in recent years and, in particular, during the pandemic.
Prima di stampare, pensa verde
Think green before you print
Testo Allegato: March 2023
Banks’ liquidity transformation rate:
by Raffaele Lenzi, Stefano Nobili, Filippo Perazzoli and Rosario Romeo
March 2023
(Research Papers)
Banks’ liquidity transformation rate:
by Raffaele Lenzi, Stefano Nobili, Filippo Perazzoli and Rosario Romeo
The views expressed in the papers are those of the authors and do not necessarily reect
Via Nazionale, 91 – 00184 Rome – Italy
ANKS
’
LIQUIDITY
TRANSFORMATION
RATE
DETERMINANTS
AND
IMPACT
ON
LENDING
by Raffaele Lenzi,* Stefano Nobili,* Filippo Perazzoli* and Rosario Romeo
Abstract
the collateral pool, banks’ characteristics, and lending activity. Our contribution is twofold. Firstly,
we analyse how banks’ characteristics correlate with their liquidity transformation rate. The main
nding is that variables relating to banks’ liquidity and efciency have an impact on their liquidity
transformation rate, though we did not nd any link with nancial soundness indicators. This
supports the view that central banks can provide liquidity and relax the collateral framework without
transformation rate correlates to credit supply. Our ndings show that the banks that pledged more
the most, suggesting that the broad range of eligible collateral in the euro area may have supported
the provision of credit to the real economy in recent years and, in particular, during the pandemic.
Keywords:
Liquidity transformation rate; Liquidity Coverage Ratio; central bank credit operations;
Il lavoro esamina l’interazione tra il liquidity coverage ratio (LCR), la composizione del collateral pool,
le caratteristiche delle banche e l’attività di prestito. Il contributo è duplice. In primo luogo, si analizza
come le caratteristiche delle banche siano correlate al loro tasso di trasformazione della liquidità . Il
risultato principale è che le variabili relative alla liquidità e all’efcienza delle banche hanno un impatto
sul loro tasso di trasformazione della liquidità , mentre non risulta alcun legame con gli indicatori di
solidità patrimoniale. L’analisi conferma, pertanto, l’idea che le banche centrali possano fornire liquiditÃ
e ampliare la gamma di garanzie idonee, senza assumere rischi eccessivi nei loro bilanci. In secondo
luogo, si esamina la correlazione tra il tasso di trasformazione della liquidità delle banche e l’offerta di
credito. Dall’indagine emerge che le banche che impegnano più attività illiquide in cambio di riserve di
banca centrale sono quelle che hanno aumentato maggiormente i loro prestiti all’economia, suggerendo
che l’ampia gamma di garanzie idonee nell’area dell’euro avrebbe supportato l’erogazione di credito
all’economia reale negli ultimi anni e, in particolare, durante la pandemia.
Introduction
The liquidity transformation rate: composition, trend and distribution
Variables and data
Panel analysis
COVID-19 period
Robustness check
Liquidity transformation rate and bank lending
4.1
The collateral relaxation measures
References
Tables and gures
7
1.
Introduction
1
The global
financial crisis
(GFC)
highlighted how important it is for banks to hold an adequate
liq
uidity buffer in order to with
stand severe short
–
term liquidity shocks.
On 1
st
October 2015 the
liquidity coverage ratio (LCR) was introduced as the first
quantitative liquidity requirement for
financial institutions
at
the European
Union
level
.
Under the LCR regulation, banks are
required to
hold a
n adequate
stock of unencumbered high
–
net cash outflows over a 30
–
day stress scenario.
Over recent years
,
cent
ral banks have conducted longer
–
term credit operations to ease
fu
nding
conditions for banks.
Between 2014 and 2019,
the
Eurosystem launched three series of
targeted
longer
–
term refinancing operations (TLTROs)
, after the introduction of two rounds of very long
–
term
refinancing o
perations
(VLTROs)
in December 2011
.
By offering
banks long
–
attractive conditions
, t
hese operations lower
ed
banksâ?? funding costs and
facilitate
d
liquidity
management
.
They
strengthen
ed
incentivizing
bank lending
to the real economy.
The outbreak of the COVID
–
19 pandemic posed great challenges to the real economy worldwide.
There was
concern
of a large wave of non
–
financial
firm
sâ??
defaults,
possibly
generating
a credit
crunch
.
The major
fear
w
as
that
banksâ?? inability or unwillingness to supply credit would
have
exacerbate
d
the effects of the pandemic. Against this background,
since March 2020
the Eurosystem
rates
and, ultimately, to preserve the flow of credit to households and firms in all sectors and countries.
The
Eurosystem
eased the conditions of the TLTRO
–
III
programme
and introduced new
LTROs
. The
TLTRO
–
III
has been a powerful tool to counter
act
credit contraction, since it directly
addressed the elevated credit requ
es
ts
from
many firms, espec
ially the
s
mall and medium
–
sized
enterprises (SMEs)
which lacked
access to capital markets. The
in
–
built incentive scheme made
it
attractive for banks to
call on
TLTRO
–
III
funding in order to extend credit to firms and households
(see Lane, 2020)
.
According to
the Bank Lending Survey (BLS)
,
the
TLTRO
–
III
programme
has supported credit
provision. Moreover, banks have indicated that
TLTRO
–
III
ha
d a net easing impact on the terms and
conditions offered to borrowers and a positive net impact on their lending volumes.
The Eurosystemâ??s
interventions
together with the contribution
o
f
Governments
,
which
implemented several policy
measures to support
credit provision, such as debt moratoria and public guarantees
,
protected
bank
–
based credit flows
.
3
1
Any views expressed in this paper are the authorsâ?? and do not necessarily represent thos
e of the Bank of Italy.
We are
grateful to
Luigi Cannari, Patrizia Ceccacci, Gioia Cellai, Alberto Locarno, Franco Panfili,
Stefano Siviero
and
one
anonymous refer
ee
for valuable comments and suggestions.
We also thank Paola
Antilici,
Stefania
Marco
Luca and
Gianluca
osconi
for comments on a
previous version of this paper
.
2
See ECB, 2020a; ECB, 2020b; ECB
,
2020c.
3
In the case of Italy, debt moratoria for SMEs were introduced by Decree Law 18/2020 (
â??
Cure Italy
â?
decree) and
measures related to new loans
backed by public guarantees
(via the Central Guarantee Fund or the public agency
SACE) by Decree Law 23/2020 (
â??
Liquidity Decree
â?
).
��8
The effectiveness of TLTRO
–
III wa
s further supported by the temporary capital relief measures
introduce
d by ECB Banking Supervision
,
the European Banking Au
thority (EBA), and the National
.
4
The
Eurosystem
also
adopted a package of temporary collateral easing measures to
increase
the
availability of eligible collateral
and
to
allow
counterparties
to
participate in liquidity providing
operations, such as the
TLTRO
–
III
.
5
The measures collectively support
ed
the provision of bank l
oans
especially by easing the condi
tions at which credit claims were
accepted as collateral. At
the same
time,
the Eurosystem
increas
ed
its risk tolerance, particularly by lowering collateral valuation haircuts
6
As part of
additional credit claims scheme (ACC)
,
Bank of Italy
included
as eligible collateral
new loans
backed by public guarantees
and
portfolios
of consumer loans
.
T
his
paper
a
ddress
es
the interaction between
LCR,
the composition of the
collateral
pool, bank
characteristics
and lending activity
.
T
here is a fundamental
relationship
between
LCR,
the
role of the
Eurosystem
as
liquidity
provider
and its collateral framework
. S
ince central bank reserves are treated
as HQLA, banks can ful
fi
l the
LCR requirement
by borrowing from the Eurosystem against eligible
non
–
HQLA
collateral
.
W
e use
the liquidity transformation rate (LTR), an indicator
recently proposed
by Hartung (2020),
to
ca
lculate
the amount of net HQLA that a
bank
generate
s
pledg
ing
specific
,
instead of keeping them
unencumbered
.
LTR can be
calculated in a granular way, a
t the level of
, by comparing the haircuts and the
Eurosystem eligibility criteria with those
enacted
in the LCR
regulation
.
It can also be
aggregated at
bank level
,
allow
ing
to compare the extent of liquidity transformation over time and
cross
–
section
.
Our empirical analysis is focus
ed on Italian banks
. For these banks, we have
calculated
the LTR
on
a monthly basis for the period from October 2015 to J
anuary 2022.
We
focus
on the time interval
following the outbreak of the pandemic.
The c
ontribution of t
his paper is two
fold.
First
ly
, w
e
analys
e
how
bank
characteristics
correlate with
th
e
LTR
. Our aim is to understand
whether
counterparties
with low (high
) f
inancial soundness have
a high
(low)
liquidity
transformation
rate
or
if
, rather, the latter is the
r
esult of an efficient liquidity
, e.g.
to be used
in
the re
po
market.
In this
regard,
we
conduct
a
panel regression
at bank level
, where the
independent
variab
les
can be classified
into
six
categories:
(i)
size
;
(ii)
efficiency
;
(iii)
liquidity
;
(iv)
capital
adequacy
;
(v)
riskiness and
(vi)
opportunity cost of participating in refinancing operations.
The main finding of our analysis is that
variables relating to
bank
V¶
liquidity and efficiency have an impact on the
ir
liquidity transformation
rate, but we
do not
find any
link
with financial soundness
indicators. Results show
the absence of a
��
4
6HHWKH(&%%DQNLQJ6XSHUYLVLRQSUHVVUHOHDVHHQWLWOHG³(&%%DQNLQJ6XSHUYLVLRQSURYLGHVWHPSRUDUFDSLWDODQG
operational
UHOLHILQUHDFWLRQWRFRURQDYLUXV´0DUFK,QDGGLWLRQVHHWKHEORJSRVWE$QGUHD(QULDHQWLWOHG
³)OH[LELOLWLQVXSHUYLVLRQKRZ(&%%DQNLQJ6XSHUYLVLRQLVFRQWULEXWLQJWRILJKWLQJWKHHFRQRPLFIDOORXWIURPWKH
FRURQDYLUXV´0DUFK
5
The range of eligible collateral can vary considerably across time and central banks; the Eurosystem
already
accepted
–
FODLPVRU³RZQXV
HG´VHFXULWLHVLHLVVXHGEDQHQWLWZLWKZKLFKWKHEDQNWKDWKROGVWKHFROODWHUDOKDVFORVHOLQNV
6
6HH/GH*XLQGRV,6FKQDEHO³,PSURYLQJIXQGLQJFRQGLWLRQVIRUWKHUHDOHFRQRPGXULQJWKH&29,’
–
19 crisis: the
(&%¶VFROODWHUDOHDVLQJPHDVXUHV´
(&%
%ORJ

DQG
35
/DQH
³7KH
PRQHWDU
SROLF
UHVSRQVH
WR
WKH

SDQGHPLFHPHUJHQF´(&%%ORJ
9
systematic credit risk
transfer
from
credit intermediaries
to the central bank
,
support
ing
the view that
central banks can provide
liquidity without absorbing undue
risks onto their balance sheet
s
.
O
ur study is closely related to Fecht
et al.
(2016)
and de Roure and
McLaren
(2020)
.
I
n
the context
of German and British banks, respectively,
they
se
ek
evidence
o
f â??
s
ystemic
a
rbitrageâ?, where riskier
banks
take up more credit from the central bank and
pledge
The former, who
use data on
German
banks from 2006 to 2010,
find evidence of this relationship, so they
argue that
the ECB collateral framework does not price the
correlation risk between counterparty and collateral,
which would otherwise be priced in
private markets.
The latter
find no evidence
o
f such an arbitra
ge
opportunity in the UK context
.
With respect to these two papers
, we broad
en the analysis by considering
other
bank
characteristics
by
tak
ing
into account the introduction of the LCR regulatory
requirement and its possible interaction with the choices of banks regarding
collateral
.
A number of papers has recently studied t
he
pledged
as collateral
with the Eurosystem and liquidity regulatory requirement. Hartung (2020)
presents
the liquidity transformation rate (LTR)
,
a
new measure
for
assetsâ??
liquidity pro
fi
le
,
a
t a level
,
at
bank
level and for
individual euro area countries
. He finds
that banks follow a
hierarchical approach
in
managing their collateral pools,
prefer
ring to pledge with the Eurosystem
first less
and
, successively,
s, such as government bo
nds
.
H
owever
, the
to verify the existence of a relation between LTR
and bank
characteristics
.
Kedan and
Ventula
Veghazy (2021)
compare banks
â??
demand for central
bank reserves before and after the introduction of
the LCR in 2015. They find
that banks with low
LCR increased their
demand for
central bank reserves
more than those with high LCR
. Schmidt
(2019) shows
that
,
in countries without
liquidity requirement prior to the intr
oduction of the LCR
,
banks have pledg
ed less liquid
collateral with the Eurosystem after
the introduction of the harmonized
requirement.
Second
ly
,
the paper investigates
how
LTR
correlates with
credit supply
, at
individual bank level. The
intuition
is that if a bank
pledges as collateral a
non
–
, both
its liquidity transformation rate and its LCR rise;
therefore
,
the bank could become
more willing to
engage in activity that c
an
reduce the LCR, such as lending to the real economy.
Our f
indings
confirm
this
hypothesis:
banks with high
LTR increase their lending to nonâ??financial firms and households
more
than th
e
o
n
e
s
with low LTR
, suggesting
that the broad range of
eligible
collateral in the euro
area may have helped the provision of credit to the real economy in recent
years
and in particular
during the pandemic.
In this way,
we
contribute to the literature
regarding t
he impact
on the real
economy
.
Gocheva
et al.
(2022
) have drawn similar findings in a recent paper
. Their
analysi
s suggests that
attractively priced central bank credit operations in which a broad range of less
li
accepted as collateral can provide strong incentives for banks to take actions that
reduce their LCRs.
Such actions
could include the provision of additional credit
.
More in general, we
also
contribute to the empirical
assessment
of the transmission of unconventional
–
19, we find that collateral relaxation
measures
may
10
as collateral, Eur
osystemâ??s measures ensured that the banking
sector retain
ed
access to ample central
bank liquidity at
favorable
terms.
Related
literature
includes
Barthélémy
et al.
(2017)
,
who
show
that an increase in the share of illiquid
with the
Eurosystem
i
s
associated with greater resilience of
banksâ??
lending activity
(
with reference to
larges
t euro area banks between 2011
and 2014
)
.
Carpinelli and Crosignani (2020)
analys
e
the transmission of the three
–
year
V
LTRO
in
Italy from December 2010 to June 2012
and
find that
the eligibility of new assets as collateral at the central bank helped banks
to
restore their
credit supply
,
by
supporting their access to
V
LTRO
.
Chan
et al.
(2018) show
that the key
driver of
the VLTROs
â?? effectiveness was the maturity extension of ECB lending to banks
in combination with
allowing banks to use loans to firms as collateral.
Choi
et al.
(2021)
build a model that incorporates
maturity transformation and collateral circulation
. They
show that
it can be optimal
,
for the central
bank
,
to lend against low
–
qua
lity collat
eral since it improves liquidity and the functioning of markets.
Other studies
that are
focus
ed
on
the impact of the
TLTROs
on the amount of credit granted to non
–
financial private
corporations
include
Esposito
e
t al.
(2020
)
,
Andreeva and
Garcia
–
Posada (2019)
.
Altavilla
et
al
.
(2020)
find
that
the TLTRO
–
III
programme
complemented by
supervisory authorit
i
es
â??
interventions
ha
s
been able to miti
gate the adverse impact of COVID
–
19 on
loans growth.
Finally,
f
or banks with the same business
model, t
he liquid
ity transformation rate
can
be considered
as
an indicator
of banksâ?? efficiency in the liquidity
management
process, measuring their ability to
m
inimize the cost of liquidity
as well
as optimiz
e
compliance with
liquidity
regulatory constraints.
High values of LTR could
suggest that banks are
willing to engage in activity supporting the real
economy, such as
the provision of loans.
In a similar vein,
Albanese and Ciocchetta
(2021) show the
importance of organizational efficiency variables in supporting credit supply. The authors find that
these variables resulted more important than banksâ?? balance sheet characteristics in explaining ba
nk
lending in Italy during the pandemic
.
The remaining part of the paper is organized as follows. Section 2 focuses on
the
LTR indicator,
analys
ing its
composition,
trend and distribution from October 2015 to January 2022
.
In s
ection
3
we
present the results of the ec
characteristics; section
4
focuses on how
the level of l
iquidity transformation rate
has an impact on
bank lending
.
Section
5
finally concludes.
2.
The liquidity transformation rate
: composition,
trend and distribution
The liquidity transformation rate
(LTR)
, introduced by Hartung (2020), indicates how much HQLA
a bank obtain
s
7
Such transformation is possible
thanks to different haircuts between
LCR regulation
and
the
Eurosystem collateral framework. In
order to calculate
the
LTR, it is necessary to derive a quantitative measure at a granular level, starting
from each individual
asset
j
at time
t
with its related haircuts (equation 1). Successively, the liquidity
transformation rate can be computed for each bank or jurisdiction
i
by aggregating the results
(equation 2).
7
The rate can be computed by using either the mobilised or the encumbered collateral. In this work, we refer to the
mobilised collateral.
11
ܮܴܶ
à¯
,
௧
=
1
â??
à¯à¯?�஺
à³?
,
�
�಴�
஼�஺à¯
à³?
,
�
�಴ಳ
=
1
â??
1
â??
â??
à³?
,
�
�಴�
1
â??
â??
à³?
,
�
�಴ಳ
=
â??
à³?
,
�
�಴�
â??
â??
à³?
,
�
�಴ಳ
1
â??
â??
à³?
,
�
�಴ಳ
(1)
ܮܴܶ
�
,
௧
=
1
â??
â??
à¯à¯?�஺
à³?
,
à³?
,
�
�಴�
à³?
â??
಴�ಲ�
à³?
,
à³?
,
�
�಴ಳ
à³?
(2)
T
he term HQLA
LCR
–
specific LCR
–
haircut
(
â??
à¯
,
௧
�஼�
)
8
,
while the term CVAH
ECB
represents the collateral value of that
ework
(
â??
à¯
,
௧
�஼஻
)
.
For instance, LTR
is generated.
We can
look over
two polar cases
in order to
better
understand the functioning of the LTR
:
i
does not generate HQLA in terms of
LCR regulation
(i.e. it has a haircut equal to 100%) but it is
accepted
as collateral
in the
policy
pool, the LTR is equal to one (numerator of the second
term is zero)
,
while if the two haircuts are i
dentical, the liquidity transformation rate is zero (the
second term is equal to one). The larger the difference between two haircuts, the higher is the liquidity
negative
since the LCR
–
haircut could be lower than the Eurosystem
–
haircut, such as for government bonds.
9
Therefore, the ratio HQLA/CVAH represents the opportunity cost of mobilising and, eventually,
–
HQLA assets with limited utilization in the financial markets. This approach allows the banking
system to make li
quidity management more efficient by minimizing liquidity costs and those
associated with the fulfilment of liquidity requirements.
Large b
anks with a diversified business
model are more likely to carry out this strategy than small or specialised banks, al
so considering the
relevance of the information
technology
system for the pledging and monitoring of credit claims in
8
The LCR is computed according to
the following formula: LCR=
Liquidity buffer
/(Outflows
–
min(Inflows;
75%×Outflows))
where outflows are calculated by multiplying the outstanding balances of various categories or types of liabilities and
off
–
balance sheet
commitments by the rates at which they are expected to run off or be drawn down in a specified
stress scenario. Inflows are calculated by multiplying the outstanding balances of various categories of contractual
receivables by the rates at which they are
expected to flow in under the scenario up to an aggregate cap of 75% of
outflows.
held in the central bank and which can be withdrawn at any time
during stress period (free central bank reserves,
include covered bonds of lower credit quality, ABS and corporate debt securities.
Each of thes
e asset categories is subject to speci
fi
c haircuts, which are summarised in Grandia
(2019) and range
from 0% for central bank reserves and central
government bonds to 50% for
eligible corporate bonds with rating
BBB+ and BBB
–
.
9
In this cas
Under
the perspective of easing the
granted by the ECB
Banking Supervision
during the pandemic, can be positively evaluated.
12
the collateral pool. As refinancing with the Eurosystem increases, it is however plausible that the
liquidity trans
formation rate will dec
rease, because
banks will encumber more liquid assets.
In periods of excess liquidity, banks register high LCR and
the
liquidity transformation process
becomes less crucial to fulfil liquidity requirements. The easing of the collateral framework
incentiv
iz
es banks to pledge credit claims that would otherwise
be unused on balance sheets in order
to obtain liquidity to expand lending. In fact, the appraisal of the loans at their nominal value allows
banks to stabilise the level of the collateral pool, espec
ially in the event of tensions in the financial
markets, when the prices of securities fall.
Figure
1
present
s the
trend of
liquidity transformation rate of the Italian banking system from October
2015 to January 2022
, which
ranges between 0
.
6
0
and 0
.
75
i
f calculated as
weighted average
10
and
between
0.34
and
0.47
if calculated as
simple
average
of LTRs
at individual bank level.
At the end
of
January 2022, liquidity transformation rate is
0.65
as weighted average and
0.47
as
simple
average
.
Figure 1
â??
LTR
time series of the Italian banking system
Source: Eurosystem and Bank of Italy
The trend of liquidity transformation rate is strictly correlated to LCR and
to the
composition of the
collateral pool.
In
this regard, i
n the same
period
the average LCR of the Italian banking system
rose
from about 150% to over 200%
11
,
against a reg
ulatory minimum of 100%
12
, despite the increase
of
the
net cash outflows
over a 30
–
day stress scenario
(denominator of the LCR;
F
igure
1
A
in A
ppendix
)
.
Such
trend is
mainly due
to the extraordinary measures promoted by the Eurosystem
to
support
liquidity; the increase in cu
stomer deposits also contributed
.
The
se measures
favoured
the increase
of t
he liquidity buffer (numerator in the LCR formula)
, which
c
onsists of approximately 90% of level
.
10
The calculus of the weighted average is obtained considering the rat
in terms of liquidity
requirements
(
–
specific LCR
–
haircut
)
mobilized
pool
by Italian
counterparties
and
the sum of collateral values of those assets (after
applying
the h
aircuts of the Eurosystem collateral
framework
)
. The value for Italian banks is in line with those of other euro area countries (see Hartung, 2020).
11
The
LCR
of the Italian banking system
is
calculate
sum of the
liquidity buffers and the
sum
cash
outflows of all Italian banks.
12
It should be noted that
,
as part of the measures
in response
to the
pandemic
, the possibility of operating temporarily
with LCR levels below 100% ha
s been introduced
.
The mini
mum LCR under the CRR was
: 60% from 1 October
2015, 70% from 1 January 2016, 80% from 1 January 2017 and 100% from 1 January 2018
.
13
In 2017 and
2018,
the liquidity buffer steadily fluctuated around an average of
â?¬
400 billion, while in
2019 it was close to
â?¬
500 billion due to an increase
in both government bonds and
central bank
reserves
. After the outbreak of the pandemic, the liquidity buffer significantly rose up to
â?¬
720 billion
in December 2021 as a result of the growing central bank reserves held by the counterparties (from
â?¬
115 billi
on in March 2020 to
â?¬
425
billion in December 2021
â??
see F
igure
2
A.a).
13
During the last two years, the composition of Italian banksâ?? liquidity buffer
has
considerably changed
on aggregate: the weight of government bonds declined from 62% to 32%, while the w
eight of central
bank reserves r
ose
from 23% to 59% (see F
igure
2
A.b).
14
A
fter
an
initial
increase
following
the
TLTRO
–
II.4
allotment
in March 2017
,
policy pool
remain
ed
substantially stable until February 2020 (
F
igure
3
A
),
at
around
â?¬
300 billion.
Successively,
in
correspondence with the increase of the recourse
by banks
following the outbreak of the COVID
–
19
15
,
there
were
two
significant rise
s
pool
in March
2020
(
â?¬
55 billion) and
in
April
2020
(
â?¬
40 billion)
.
I
n the first
case,
the increase is
mainly
due
to the encumbrance
of government bonds
, while in the second case it is attributable to
the
extraordinary measures adopted to ease
the eligibility requirements
and the risk
–
control
criteria
.
16
More specifically
, th
e haircuts
–
reduction result
ed
in an automatic
rise
in the value of the collateral of
approximately
â?¬
36 billion
.
The extension of the
A
dditional Credit Claims
(ACC) by the Bank of Italy
le
d
d
adapted their
internal procedures; in a
ddition, the new measures help
ed
to incentivize 15 count
erparties to start
pledging bank loans
.
In correspondence with the TLTRO
–
III
.7
allotment
in March 2021
17
, the
d
a new p
eak
at around
â?¬
500 billion. After an initial pledge of
government bonds, the counterparties partially replace
d
th
is
type of
with bank loans
, which
consequently bec
a
me the most representative asset class of the collateral pool. Most of the bank loans
were
pledged under the
ACC
temporary scheme.
From March 2020 to
January
202
2
,
weighted average
LTR raise
d
from 0.61 to
0.6
5
(
simple
average
from
0.37
to
0.47
)
.
Albeit the increment in April 2020
wa
s due to the reduction of haircuts, in the
following months the increasing LTR tells us how banks have modified the composition of the
s in terms of
LCR regulation
.
13
It should also be noted that the two
–
tier system (TTS) introduced in October 2019 ex
empt
a part
of banksâ?? holdings
of exce
ss liquidity from the negative deposit facility rate
(currently equal to
–
0.50%)
. The maximum
amount of the
reserves that are exempt
ed
is six times the minimum reserve requirement for each bank; the remuneration rate
for the
exempt tier is zero per cent.
14
In the pe
riod January 2017
–
December 2021
available government bonds and
central bank reserves
represent
,
on
average
,
87% of the liquidity buffer.
15
The
rise was facilitated by both
the easing of the conditions of TLTRO
–
III programme and additional LTROs
, carried
out in response to the pandemic
.
16
In March 2020, the
Eurosystem
reduced haircuts o
f
.
At the same time, it decided to
decrease both the
additional
haircuts applied to covered bank bonds allocated in own
use and the
haircuts for securities valued at
A further reduction in haircuts was also applied, on a
permanent basis, only to bank loans. The Bank of Italy has extended its additi
on
al credit claims scheme (ACC),
including, from 25 May 2020, loans covered
by public guarantees issued by
the public agency
SACE and the
Central
Guarantee Fund for
SME
s on the basis of
Decree Law
23/2020 (â??L
iquidity decreeâ?).
From 17 June 2020, Italian
coun
terparties can also pledge portfolios of consumer loans as collateral
.
17
The
TLTRO
–
â?¬
74 b
illion
.
14
Figure
2
illustrates the box
–
plot of the LTR time series, which represents
for each month
the LTR
distribution of the counterparties, dividing it in four quartiles.
W
ith the exception of the
period
following the outbreak of
the pandemic
, about 25% of Italian banks record negative LTRs and 50%
of them rarely exceed the value of 0.4, while the LTRs of the last quartile are usually between 0.75
and 1.
With reference to
the entire sample period
,
the
monthly average of the
median
(
0.39
)
is lower
than t
hat of the aggregate
LTR
(0.64)
18
, therefore
we can affirm that large banks tend to record high
LTR while small and medium
–
sized banks are likely to be included in the first two quartiles of the
–
spectrum
in
cluding
â??own usedâ?
–
backed securities
(
issued by an entity with which the
bank that holds
, can generate much more liquidity transformation, while
small banks mainly
manage
government bonds that have a nega
tive LTR.
Figure 2
â??
Box
–
plot
of LTR time series
Source: Eurosystem and Bank of Italy
Note: in the figure the light
–
blue rectangles represent the two central quartiles of the distribution (25%
–
50%
and 50%
–
75%) while the horizontal bar tha
t separates
them is the
value of the median. The lower and upper
bars
represent the min
–
25% quartile and the 75%
–
max quartile, respectively.
In April 2020, the extraordinary measures that relaxed the
collateral
eligibility requirements and risk
control criteria had a
n immediate effect on the LTR distribution with a considerable increase of its
mean from 0.61 in April 2020 to 0.66 in May 2020. In particular, the reduction of haircuts determined
a raise in the value of the collateral pool even though its composition rem
ained unchanged. Also in
the following months, the LTR distribution showed some variations; the first and second quartiles are
noteworthy since their upper bounds increased until their historical maximum values
,
respectively
0.20
in November 2020 and 0.
51
in September 2020
.
The effects of the interventions
adopted by Bank of Italy and the European Central Bank
(ECB) after
the
outbreak of C
OVID
–
19
are evident by dividing the distribution of the LTR into two intervals.
From October 2015 to March 2020, the
upper bound of the first quartile remains at values close to 0
,
18
average.
��15
while, starting from April 2020, it gradually increases until a level above 0.15. The
median
, in the
last 1
6
months, remain
s
at
its
maximum values of the historical series as well.
3.
Empirical
3
.1
Variables and data
characteristics contribute mo
the liquidity transformation rate (LTR).
According to the literature (see Fecht
et al.
, 2016, and de Roure and Mc Laren, 2020), we divide the
variables identified to describe bank characteristics into
six
categories: size, efficiency, liquidity,
financial health, riskiness and opportunity cost of
participating in central bank credit operations.
We consider the size as a qualitative variable, which groups banks into four classes based on total
19
For
the
efficiency category, we use
both
and a proxy of
bank digitalization. The former is
B
anks
with
high ROA
s
should
achieve
considerable
LTR
s
.
For the latter,
we
use
a proxy
based on
EDQNV¶
expenses
for information technology (IT) adoption
.
Indeed,
Branzoli
et al.
(2021)
show that
bank
IT costs
are
positively correlated with both a higher likelihood
of offering digital services and
engaging in innovative processes
. Following these authors,
w
HPHDVXUHEDQNV¶OHYHORI,7DGRSWLRQ
using
yearly
costs for the automatic processing of data
,
reported
LQEDQNV¶LQFRPHVWDWHPHQWV
.
These
costs include
expense
s incurred for
the purchase of hardware
and software,
gross wages paid to IT
specialists
and
the
outsourcing
of IT services to external providers.
We
normalize these costs by
bank
s
¶
total
asset
s
.
The conjecture is that
banks with higher IT costs
should be more efficient in
the
liquidity management process
compared to the others
,
also considering t
he relevance of the IT
system
for the pledging and monitoring of credit claims in the collateral pool
.
As liquidity explanatory variables, we consider several indicators in order to capture the peculiarities
of the funding structure of Italian banks: LCR,
a
encumbrance,
a
strength
index on the bond
market and the net debt position on the repo market.
As described in the previous paragraph,
LCR is
a coverage
index that aims at ensuring that an intermediary maintains an adequate stock of available
ral liquidity de
ficit and banks
have a LCR
close to the regulatory threshold, banks would have greater incentives to carry out the
to
the central bank collateral pool. The
high
operating costs
associated with the mobilization toward central bank of these activities would
be more than offset by the missed cost of violating the regulatory requirement. In this context, a
negative relationship between LCR and LTR
is plausible
. However
, in the period
considered in our
analysis almost all banks have
recorded
, on average, high LCR
s
(
much larger than the regulatory
��
19
;
(2) banks with
a value of total assets between 3,626 and 21,532 billion; (3) banks with a value of total assets between 21,532 and
182,052
billion; (4) banks with total assets greater than 182,052 billion
.
16
threshold
)
incentive to minimize the use of HQLA in
central bank
credit operations has been low
.
Eurosystem refinancing over total assets shows how much of a bankâ??s balance sheet is financed by
the central bank.
Banks that have a high
refinancing
ratio should have low
LTRs. This result would
be in line with that
one found by
Hartung (2020). The author
finds that if banks want to take up more
central bank credit, they pledge additional collateral, which may differ from
th
at
previous
ly
used. The
banks, on average, follow
a hierar
chical approach, mobiliz
ing first
less
; o
nly at a later
stage
and
when
their recourse to refinancing operations
rise
, they
pledge with the Eurosystem
more
government bonds. This implies
a reduction of
the liquid
ity transformation rate
.
unding
transactions, but the second one considers only the
They can be computed according to the
following formulas:
ܣܧ
=
ܧÝ?Ü¿Ý?Ý?ܾÝÝ?ÝÝ?
ܽÝÝÝÝÝ
+
Ü¿Ý?Ý?Ý?ܽÝÝÝ?ܽÝ?
Ý?ÝÜ¿Ý�Ý?ÝÝ?
ܽÝ?Ý?
Ý?ÝÝ?ÝÝÝ?
ܶÝ?ÝܽÝ?
ܽÝÝÝÝÝ
+
ܶÝ?ÝܽÝ?
Ü¿Ý?Ý?Ý?ܽÝÝÝ?ܽÝ?
Ý?ÝÜ¿Ý�
Ý?
ÝÝ?
ܣܧܧ
=
ܧÝ?Ü¿Ý?Ý?ܾÝÝ?ÝÝ?
ÝÝ?�Ý?�ܾÝ?Ý
ܽÝÝÝÝÝ
+
ÝÝ?�Ý?�Ý?Ý
Ü¿Ý?Ý?Ý?ܽÝÝÝ?ܽÝ?
Ý?ÝÜ¿Ý�Ý?ÝÝ?
ܽÝ?Ý?
Ý?ÝÝ?ÝÝÝ?
ܶÝ?ÝܽÝ?
ÝÝ?�Ý?�ܾÝ?Ý
ܽÝÝÝÝÝ
+
ܶÝ?ÝܽÝ?
ÝÝ?�Ý?�ܾÝ?Ý
Ü¿Ý?Ý?Ý?ܽÝÝÝ?ܽÝ?
Ý?ÝÜ¿Ý�Ý?ÝÝ?
From the li
quidity risk point of view, the analysis of the encumbrance is important since the scarce
availability of unencumbered collater
al means that the bank has low
financing capacity in stressful
situations. The relationship between AE and LTR should be positive: banks
that have high levels of
AE use to
pledge
which determine high
LTR
s
.
policy)
20
, high values indic
ate that the
,
such as government bonds
,
are more used
than the others
. Th
is implies
that
, on average,
high
AEE
should
correspond
to low LTR.
Then, we consider a variable
related to
the
variation
of
sight deposits
, which is calculated as
a
weighted average of
deposits
â??
monthly changes during the previous 12 m
onths, with a
greater weight
(
w
s
) for the most recent data.
ܵ�Ý?
â??
Ý
Ý?ÝÝ?Ý?Ý�ÝÝ
Ü¿
â??
ܽÝ?Ý?Ý
=
â??
â??
Î?
Ý�Ý?
â??
Ý
Ý?ÝÝ?Ý?Ý�ÝÝ
௧
â??
௦
â??
Ý?
௦
11
௦
=
0
�
௧
=
1
Ý?
௦
=
Ý
௦
,
Ý
=
0
.
9
20
It should also be borne in mind that the
AEE ratio
is affected by the difficulty of classifying loans among
available
asse
in fact, loans
are usually co
nsidered by the reporting banks as not eligible until
they are
pledg
ed in the
policy pool. For example, consider two banks A and B, which have the same balance
sheet composition: 100 million of government bonds and 100 million of loans and th
e sam
e amount of encumbered
(equal to 150 million).
In particular, bank A has encumbered
to
the monetary policy collateral pool 50 million
of government bonds, 50 million of loans and 50 million of loans as underlying of covered bonds pledged as collater
al
s. In this case,
its AEE is
100%, since l
oans are not considered eligible assets until they are pledged.
17
This allows to capture their temporal evolution
, obtaining a proxy of
the depositors’ confidence level
toward a bank.
We compute t
he
strength
index on the bond market as the difference between net issues
21
and bonds
falling due in the following 12 months
, where both the measures are reported on a monthly base,
over
total out
standing bond
s:
ܵÝÝ?ÝÝ?Ý?Ý
â??
�Ý?Ý?ÝÝ?
Ý?Ý?
Ý
â??
Ý
ܾÝ?Ý?Ý?
Ý?ܽÝ?Ý?ÝÝ
=
Ý?ÝÝ
�ÝÝÝ?ÝÝ
â??
Ý?ܽÝÝ?Ý?�Ý?Ý?
ܾÝ?Ý?Ý?Ý
ÝÝ?ÝܽÝ?
Ý?Ý?ÝÝÝܽÝ?Ý?�Ý?Ý?
ܾÝ?Ý?Ý?Ý
L
ow
values of the indicator
denote
banks that
have greater difficulties in
raising
funding
on the bond
market.
When for a bank these
two
latter indicators (variation of sight deposit indicator and
strength
index on
pool rises, with a
consequent increase in its LTR.
The net debt position on the repo market is given by the difference between the repo positions
(liquidity obtained by the bank against collateral on the market) and the reverse repo positions
(liquidity provided by the bank
in exchange for collateral). The increase in the net debt position,
which implies a greater use of government bonds as collateral on the repo market, should correspond
to a rise in the LTR.
Ratio and Total
C
apital Ratio)
,
while b
anksâ?? riskiness is measured by their Non
–
Performing Loan (NPL) Ratio. The latter provides a
measure of the write
–
downs on loans that banks
may
face, and which will have future
effects on their
profitability and capital ratios. A negative (positive) and significant relationship betwee
n capital ratios
(NPL ratio) and LTR
would
prove
the existence of a “systemic arbit
rage”
,
in which riskier banks
pledge with the Eurosystem
less li
22
We calculate the opportunity cost of participating in central bank refinancing operations as the
difference between the yield on unsecured Italian bank bonds on the secondary market and the cost
of Eurosystem refinancing (taking i
nto account the interest rate of the TLTRO
s
). T
he greater this
difference
, the
more advantageous
for banks to participate in refinancing operations rather than
issuing bonds.
policy collateral pool to
take into account the changes in LTR that occur automatically in correspondence with changes in the
, e.g.
the
easing
of
the risk control framework
by the
ECB
occurred
in April 2020
after the out
break of the COV
ID
–
19
.
21
22
Nyborgâ??s (2016) study of central bank collateral frameworks
introduced t
he
concept
of systemic arbitrage
.
See
Nyborg
(2015) for
a summary. Fecht
et al.
(2016) resumed it.
18
I
n the annex
,
Table 1A reports
some summary
statistics for each of
the variables used, while T
able
2A shows the
ir
correlation matrix
.
From the anal
ysis of the correlation matrix
, it
results that, as
expected, AE and AEE
are correlated
, while other variables show
low
pairwise
correlation.
The sources of bank
–
level data are
both supervisory reports harmonized at European level, i.e.
Common Reporting (COREP, containing information on capital adequacy and
risk
–
specific
info
rmation) and
Financial Reporting (FINREP, which includes balance sheet items and detailed
as well as
other
datasets from
supervisory
reporting
.
23
Monetary policy reportin
g
provides
both
the
composition of the collateral pool pledged by each
Italian
bank with the Eurosystem
and
the volume
of refinancing operations of each bank.
The dataset
includes
the composition of each pool at the
security or loan level.
Regarding the refinancing operations, w
e construct a monthly series of the
stock of refinancing of each bank, taking into account
early
repa
y
ments
.
We also use data from MTS
Repo and market data from Bloomberg.
is based on monthly
data
for
the period from
October 2015 to
January
2022
.
24
October 2015
represents the date of entry into force of the LCR requirement
at
the European
Union level
.
The data
set
comprises
89
banks
the
Italian
banking system. They
represent the
complete
set of counterparties that
pledge collateral
to
the monetary policy pool
.
25
3
.2
Panel analysi
s
This section
analys
es the relationship between the liquidity transformation rate and the variables that
summarize bank
s
â?? characteristics. Our aim is to understand whether there are systemic incentives for
some counterparties to use specific
collateral assets
rather than
others. In this
regard, we conduct
panel regressions
on the data described in the previous
section
. The model specification is
the
following
:
ܮܴܶ
�
,
௧
=
â?
�
+
ß?
1
Ý�Ý?Ý
�
,
௧
â??
1
+
ß?
2
ܴܱܣ
�
,
௧
â??
1
+
ß?
3
�ܶ
ÝÝ?Ý?ÝÝ?ÝÝÝ
ܶÝ?ÝܽÝ?
Ü£ÝÝÝÝ
�
,
௧
â??
1
+
ß?
4
ܮܥܴ
�
,
௧
â??
1
+
ß?
5
ܧܥܤ
Ü´ÝÝ?�Ý?ܽÝ?Ü¿�Ý?Ý?
ܶÝ?ÝܽÝ?
Ü£ÝÝÝÝ
�
,
௧
â??
1
+
ß?
6
ܣܧ
�
,
௧
â??
1
+
ß?
7
ܣܧܧ
�
,
௧
â??
1
+
ß?
8
ܵ�Ý?
â??
Ý
Ý?ÝÝ?Ý?Ý�Ý
Ü¿
â??
ܽÝ?Ý?Ý
�
,
௧
â??
1
+
ß?
9
ܨÝ?ܽÝ?�Ý?�ÝÝ?
�Ý?Ý?ÝÝ?
ܾÝ?Ý?Ý?
Ý?ܽÝ?Ý?ÝÝ
�
,
௧
â??
1
+
ß?
10
ܰÝÝ
Ý?ÝܾÝ
Ý?Ý?Ý�Ý�Ý?Ý?
ܯܶܵ
Ü´ÝÝ?Ý?
ܶÝ?ÝܽÝ?
Ü£ÝÝÝÝ
�
,
௧
â??
1
+
ß?
11
ܥܧܶ
1
ܴܽÝ�Ý?
�
,
௧
â??
1
+
ß?
12
ܰܲܮ
ܴܽÝ�Ý?
�
,
௧
â??
1
+
ß?
13
ܱÝ?Ý?Ý?Ý?ÝÝ?Ý?�ÝÝ?
Ü¥Ý?ÝÝ
௧
â??
1
+
ß?
14
Ü£Ý?ÝÝ?ܽÝ?Ý
â??
ܽ�Ý?Ü¿Ý?Ý
௧
â??
1
+
�
�
,
௧
All explanatory variables are lagged. The term
â?
�
represents the fixed
effect at the level of single
bank, invariant over
time.
T
able
3A
reports t
he results. The first
two columns of the table show
the coefficients of the linear
panel regression
with fixed effects at bank level, where
the t
–
statistics
is
calculated based on standard
23
We use
banks
data at the highest degree of consolidation.
24
25
Only Cassa
Depositi e Prestiti and Poste Italiane are excluded from the analysis.
19
errors cluster
ed
at bank level.
26
Column 2 shows the results of the model by adding the fixed temporal
effects to take into account
events
that have an impact o
n the LTR and that are not invariant
over
time.
Since the dependent variable LTR follows a mixed distribution, where there are se
veral observations
that take values
equal to
1 and a continuous distribution for values less than 1,
Tobit
models can be a
valid est
imation approach. Therefore,
column 3
reports
the coefficients of the panel
Tobit
regression;
specifically
,
we apply
the
Mundlak
–
Chamberlain correction (Mundlak, 1978, and Chamberlain
,
1984), as proposed by Wo
oldridge (2013), which allows for
accommodating
the correlation
between
the unobserved effects and the explanatory variables.
27
In a second specification of the model
, we eliminate the variable AEE, given its correlation with the
variable AE.
With the
aim
t
o take into account the existence of any non
–
linearity in the relationship
with the LTR
, we proceed
in the following way
: i)
we add
the squared LCR
variable
; ii)
we
inter
act
equal to
1 if the size of
4A
, where in the first three
columns
we exclude all the non
–
significan
t variables,
except for
capital ratios
and the NPL ratio.
In
this way, we can test if
the capital ratios and the NPL ratio
eventually become
significant
when the
specification of the model
varies
.
Finally, the fourth column of T
able
4A
repo
rts the panel
To
bit
regression without
the
capital
ratio
variable
.
Tables 3
A
and 4
A
show that large
banks have high
er
liquidity transformation rate;
the coefficient
of
ROA,
which is a measure of the bank efficiency,
is positive
and significant
in the
Tobit
panel
regressions, indicating that as
banks
improve efficiency
, the
ir
LTR
s
increase
.
This result is also
confirmed by the positive and significant
coefficient of
IT
expenses
in the
Tobit panel regressions
.
This confirms the conjecture that more digitalized banks (as measured by our proxy based on IT
Banks with high
LCR show substantial liquidity transformation rate, but
we find a negative a
nd
significant coefficient
for the
s
quared LCR variable (see Table 4
A
). These results
suggest
a concave
non
–
linear relationshi
p between LCR and LTR:
this finding
implies that banks with liquidity ratios
close to the regulatory threshold
are not more â??aggressiveâ?
than the other banks
in exploiting the
differences between
the haircuts
and the eligibility criteria of
the Eurosystem
collateral framework
and those laid down in the LCR regulation.
28
As a
caveat
, it should be emphasized that in
recent
years
almost all banks have recorded
LCRs
much higher than the regulatory threshold.
We find that
banks
with high
ave low
LTR,
confirming
the
findings
by
Hartung (2020), according to
which
banks mob
ilize collateral with the
26
Statistical significance at the 1%, 5% and 10% levels is indicated by ***, **, and * respectively.
27
The Mundlak tes
t conducted on Tobit regression
rejects the null hypothesis of th
e absence
fixed effects at
bank
level
, invariant over time, and explanatory variables. This result recommends using the panel
Tobit
function (which
in statistical software packages, as Stata,
is applicable only for the case of random
effects) with
the Mundlak
–
Chamberlain correc
tion as suggested by Wooldridge, which allows for
loosen
ing
the restrictive
hypotheses of random effects
.
28
the variable
log(LCR
–
regulatory
minimum)
. This variable allows to capture
banks with liquidity ratios close to
the regulatory threshold are
more
or less
â??aggressiveâ? than the
other banks
.
The results, a
vailable on request, confirm that these banks have not higher LTRs than the o
thers.
20
central bank
following a hierarchical approach:
To verify
whether this result is al
ways true, even for small
–
sized banks,
we examine the existence of non
–
linearity in the relationship between Eurosystem refinancing and LTR.
The Eurosystem refinancing
over total assets is interacted with a dummy variable that assumes value 1 if
bank’s ass
ets are
less
than the median.
The results show that the coefficient is negativ
e and significant for the large
banks,
while it is positive and significant (in the
Tobit
panel regressions
) for the small
banks.
The presence
of fixed operating costs for loansâ??
mobilization and subsequent monitoring, including those incurred
to install adequate information technology systems, influences t
he propensity of counterpartie
s to use
them
as collateral with the central bank
. Therefore, small
banks will be willing to bea
r these costs
only if their ratio between refinancing and total assets increases.
:
b
anks
that have
, either as underlying as
sets for securitization and
covered bond issues or as collateral with the Eurosystem.
As expected, the relationship between AEE and LTR is negative. Given that the AEE is calculated
), a high
value indicates that very
more
than the others, resulting in low LTRs.
Banks that have greater difficulties in borrowing on the bond market (i
.e. measured by low values of
the
strength
bond market indicator) have an incentive to take up credit from the central bank.
If they
face difficulties in issuing bonds, they will be incentivized to find alternative funding, and taking
action to use
,
as col
lateral with the central bank
,
,
we find a negative and
significant coefficient
in the linear panel regressions;
negative but not significant in the
Tobit
panel
regressions.
The coefficients of the sight deposit changes as w
ell as
those
of the net debt position on repo market
are not significant.
Turning to the
variables linked to banksâ?? financial health and riskiness
,
we do not find significant
relationship
s
in
almost all
specifications
.
financial health, the
relationship with the LTR is not significant.
As robustness checks,
we also use
the TIER1 Ratio and
Total Capital Ratio,
obtaining similar
results.
With regard to the relationship between NPL Ratio and
LTR,
Table 3A
shows that the co
efficient is not signi
ficant; on the
contrary, T
able 4A
reports a
negative and significant relationship in the
Tobit
panel regressions once the other no
t
–
significant
variables have been eliminated.
While this evidence is
suggestive of the absence of a systematic
transfer of credit risk from the private sector to the central bank, we also conducted a further analysis
ollateral pool to confirm that
widening the range of eligib
le
co
llateral does not imply the absorp
tion of undue risk in central bank’s balance sheet.
In this sense,
we assessed
looking forward
the riskiness of pledged
The analysis shows
that
the rating of
marketable
pledged in the collateral pool
by
Italian banks remained
substantially unchanged
,
on average
,
from year
t
to year
t+1
,
over the period 2015
–
202
2
.
These findings support the idea that
central banks can provide liquidity, even against a wider range of eligible collateral, without
21
absorbing u
ndue risk in their balance sheets. Offering this form of liquidity transformation can help
improve liquidity in financial markets, preserving financial stability as well.
and the LTR
is negative: reductions in haircuts, such as those that occurred in April
2020 to tackle the pandemic,
le
d to an automatic increase in LTR for all banks.
3
.3
COVID
–
19 period
The aim of this subsection is to answer the following questions:
d
id
the impact on the liquidity
transformation rate of variables relating to bank efficiency and liquidity diminish after the COVID
–
19 outbreak?
D
id
the effect of the financial health
and the riskiness
variables change?
First
ly
, we
ru
n
panel regressions
with bank
fixed effects
and
standard errors clustered
at the bank
level
over
the period following the outbreak of the
COVID
–
19 pandemic
(from
March
2020 to January
2022)
.
The results, reported in Table 5A
, are broadly consistent with the hypothesis that
also in the
COVID
–
19
period banks in better liquidity and efficiency conditions have higher liquidity
transformation rate, with the same degree
of statistical significance as
in
the previous section.
T
he
coefficient is positive but not significant,
confirming that banks with low
capital
ratios
do
not carry out more
liquidity
transformation.
The
NPL ratio shows a positive and significant
coefficient in case of panel
Tobit
regressions.
29
Second
ly
, a
s a robu
stness
check, to
the estimates on the entire sample
we add
variables
interacti
ng
with a dummy
that take the
value
equal to
1 in correspondence with the period following the spread
of
COVID
–
19
and zero otherwise
. In this way, we can better appreciate the differences in the
significance of the variables.
T
he results ar
e substantially confirmed. T
able
6A
shows that over
the
COVID
–
19 period the coefficient on
the variable associated with the
is
positive a
nd
significant
in almost all regressions
.
This
shows that over the COVID
–
19 period
the
finding of the
absence
of
systematic credit risk
transfer
fr
om counterparties to
central bank
s
is
strengthened
.
Furthermore,
we also find that the
coefficients
related to
efficiency
variables
(
ROA
, IT expenses)
are
positive
and
significant
in
Tobit
regressions
, which
may reflect
the fact
that banks with
both
high
ROA
and IT expenses
use
d
as collateral
more efficiently
during
the
p
andemic
.
3.4
Robustness check
As highlighted in the previous subparagraphs, w
e consider
in the estimates
as explanatory variable
the average haircut of the mo
netary policy collateral pool in order to
take into account the changes in
LTR that occur automatically in
correspondence with changes in the monetary policy collateral
haircuts
. However, this could not be sufficient to
fully separate the impact of bank characteristics on
29
On
average, in recent period the riskiness of Italian banks, measured by the NPL Ratio, diminished compared to
previous years, also thanks to NPLsâ?? massive disposals.
22
LTR from that of contemporaneous changes in the ECB haircut policy (holding bank character
istics
constant).
To fully dispel this concern, we run a robustness analysis
on
the
sub
–
period
from February 2017 to
February 2020,
where the ECB collateral framework was
substantially
constant
.
Specifically
,
t
he
haircut policy of the Eurosystem
collateral
framework
from
October
2015 to
January
2022 has been
changed only twice: one at the beginning of 2017 and another in April 2020.
R
esults, reported in
Ta
ble 7
A,
confirm
the hypothesis that banks in better liquidity conditions have higher liquidi
ty
transformation rate,
while
banks with low capital ratios do not carry out more liquidity
tran
sformation.
Furthermore, the NPL ratio coefficient is negative and significant.
4
Liquidity transformation rate and bank lending
4.1
credit supply
Loans to
non
–
financial
sector
have been
risin
g
over
the entire
sample
period
and
in particular after
the outbreak of COVID
–
19
(Figure
3
)
.
Figure 3
â??
Credit dynamics, Eurosystem r
efinancing and LTR
Source:
Eurosystem and
Bank of Italy.
(*)
Simple average.
It is likely
that the composition of the collateral pools pledged with the central bank
and its interaction
with
the criteria laid down in the
LCR regulation
matter for the supply of bank loans to the economy
.
W
e are interested in determining whether the liquidity transformation rate is
not
neutral on banksâ??
lending
behavior
.
We
can identify
at least
four
reasons
, already appeared in previous papers
(i.e.
Barthélémy
et al.
, 2017,
Gocheva
et al.
, 2022
)
, supporting
this conjecture
.
Barthélémy
et al.
(2017)
show that, in periods of market tensions,
a
bank with a low liquidity
transformation rate (a collateral pool mainly
composed
) is
more exposed to
s
as collateral d
rop
s
. This is
the same mechanism highlighted by Kiyotaki and Moore (1997), and defined as financial accelerator.
By contrast, a bank with high LTR p
ledges in the collateral pool also nonâ??marketable assets and so it
23
is more insured against price variations, given that credit claims are less proâ??cyclical since their
valuation only depends on their default probability.
The intuition is that, in periods o
f market tensions,
a bank with a collateral pool less exposed to market fluctuations has a greater propensity to take
actions such as the provisions of
loans, compared to the other banks
.
Furthermore
,
when
easing the conditions at which credit claims
a
re
accepted as collateral
, the central
bank is relaxing the borrowing constraint of banks
(Ahn
et al.
, 2016); therefore,
banks
become
more
willing to grant loans to the real economy. S
ince
selling credit cl
aims is costly and lengthy
,
as
this
requires securitizing them in the form of assetâ??backed securities or covered bonds
, it results
relatively
simpler
posting
them as
collateral for central bank refinancing operations
. On the contrary
,
have
the
alternative
to be used
a
lso as repo
on the securitized interbank market or
to be
sold quickly.
P
ledging
credit claims has an
opportunity cost
lower than that associated to
marketable securities.
Third
, when a bank receives credit from the central bank
using
non
–
as
collateral, both
its
LTR and LCR increase.
Our hypothesis is that
in this case
bank
s
beco
me more willing to engage
i
n activity that could reduce their
LCR, such as lending to the real economy
.
In this regard
, we follow
Gocheva
et al.
(2022
), whose
analysis, focused on the euro area after the onset of the CO
VID
–
19,
shows that banks that ge
t strong LCR boosts from ce
ntral bank credit tend to
increase
the provision
of lending to the real economy.
Finally, for banks with the same business model, the liq
uidity transformation rate can be considered
as an indicator of
banksâ??
efficiency in the
liquidity management
process
,
i
.e.
minimizing the cost of
liquidity as well as optimizing compliance with regulatory constraints. H
igh values of
LTR could
thus
suggest
that banks
a
re
proactiv
e
in supporting
the real economy
through the provision of loans.
Albanese and Ciocchetta (2021) show the
importance
in supporting credit supply
of organizational efficiency
variables
,
such as
the use of
to assess the prospective borrowers
â??
credit risk
.
The autho
rs find that these variables
resulted more
important than banksâ?? ba
lance sheet characteristics in explaining bank lending in Italy during the
COVID
–
19.
To test these hypothese
s on our sample of
87
Italian
banks
30
from
October 2015
to
January 2022
, we
regress t
he loans to
the
nonâ??financial sector
(households and nonâ??financial corporations)
31
on the
liquidity transformation rate
. We are primarily interested in determining whether
the coefficient of
this variable
is significantly greater than zero, i.e. whether a bankâ??s ability to
realize high value
of
LTR
i
s associated with
its lending to the economy.
We estimate the following
panel
equation:
30
C
ompared to the analysis of the previous paragraph
,
we exclude two
banks
with insufficient observations in the
.
31
In particular, we consider
l
ogarithm of the total outstanding loans to
nonâ??
financial corporations and households
,
excluding loans for house purchase purposes
.
24
Ü®Ý?Ý?
Ý?Ý?ܽÝ?Ý
�
,
௧
=
â?
�
+
ß?
1
ܮܴܶ
�
,
௧
â??
1
+
ß?
2
Ü®Ý?Ý?Ü´ÝÝ?�Ý?ܽÝ?Ü¿�Ý?Ý?
�
,
௧
â??
1
+
ß?
3
ܥܧܶ
1
ܴܽÝ�Ý?
�
,
௧
â??
1
+
ß?
4
ܰܲܮ
ܴܽÝ�Ý?
�
,
௧
â??
1
+
ß?
5
â??
ܩܦܲ
௧
+
ß?
6
ܮܴܶ
�
,
௧
â??
1
Ã?
â??
ܩܦܲ
௧
+
�
�
,
௧
All explanatory variables are lagged
, except macroeconomic variables
. The term
â?
�
represents the
fixed
effect at the level of single bank, invariant over time.
The coefficient
ß?
1
measures to what extent
bank
s
with high
LTR
s
expand
thei
r lending more than those with low LTRs
. The coefficient
ß?
2
assesses whether
the
level of Eurosystemâ??s credit obtained by
a bank p
lays an additional role in
deter
mining lending decisions.
We also
consider
the quality of ba
nks by
atio
and the NPL Ratio
.
We control for
contemporaneous loan demand
and
, more in general,
the co
–
movements of supply and demand by adding macroeconomic controls
to the analysis (
change
s
in
Italian
GDP
,
industrial production and
business
confidence)
as wel
l as their interaction with LTR
32
.
Residuals are clustered at the bank level to allow for heterogeneity in the distribution of shocks.
To
account for the inertia in loan creation, we also include
in one specification of the model
the lagg
ed
dependent variable as an explanatory variable.
We estimate the equation both on the entire sample and on the period followin
g the COVID
–
19
outbreak. Table
s
8
A
(based on entire period) and 9A (based on the sub
–
period February 2020
â??
January 2022)
report the regression estimates in which we introduce one explanatory variable after
another.
The results show that a
high
LTR is associated with a significant
increase
in loans to the
economy
,
both
in
the
entire sample and
in
the COVID
–
19 period. We also
find a positive relation
between the total volume of Eurosystemâ??s credit obtained by a bank and
its granted
loans;
specifically, the correlation is significant in the COVID
–
19 period.
Banks that pledge more illiquid
those that increased lending to the economy the most.
In the entire sample,
v
ariables included
to consider
do not have statistically significant coefficients.
Macroeconomic variables have positive and
statistically significant coefficients: a growth of these variables is related to an expansion of granted
loans, as expected. The interaction with LTR is significant only for the industrial production variable,
whi
le the LTR
variable
remains significant
in all the specifications
. In this way, we
can exclude that
the increase in loans by
high LTR banks is driven
solely
and fully
by the demand side of the market
.
To exploit better
the relation between loans and LTR for bank
–
month period observations with
positive liquidity uptake from the Eurosystem, we
also
estimate the Heckman selection model.
This
approach
combines a selection mechanism
focused on
positive
Eurosystem
liquidity up
take
s
with a
panel regression of
loans
on the
LTR
and the
time
fixed effects.
We also control for the quality of
ba
atio
and the NPL Ratio
.
As before, r
esiduals are clustered at the bank
level to allow for heterogeneity in the dis
tribution of shocks at the bank level
.
The selection equation
is
:
32
I
GDP
data
are at constant price, seasonally adjusted; industrial production is a constant price value index,
seasonally adjusted and the business confidence is an indicator seasonally adjusted. The source of data is
the
Italian
National
Institute
of
Statistics
(ISTAT)
and data are obtained by Refinitv Datastream. Q
uarterly
Italian
GDP
data are
reported on a monthly basis by appl
ying the cubic spline technique.
25
Ý?
�
,
௧
=
ß?
â?²
Ý?
�
,
௧
+
Ý?
�
,
௧
The regression model is:
Ý?
�
,
௧
=
ß?
â?²
Ý?
�
,
௧
+
�
�
,
௧
e
stima
t
ed if
Ý?
�
,
௧
=
1
where
(
Ý?
�
,
௧
,
�
�
,
௧
)
are assumed to be bivariate normal [0, 0, 1,
�
�
,
�
].
The variable
Ý?
�
,
௧
takes value
equal to
1
if
Ý?
�
,
௧
â??
>
0
and equal to
0 if
Ý?
�
,
௧
â??
=
0
, with
Prob
(
Ý?
�
,
௧
=
1
) =
Φ
(
ß?
â?²
Ý?
�
,
௧
)
e
Prob
(
Ý?
�
,
௧
=
0
) =
1
â??
Φ
(
ß?
â?²
Ý?
�
,
௧
)
,
respectively.
Variable
Ý?
�
,
௧
=
1
indicates
that the bank
i
on time
t
has positive liquidity uptake (takes credit)
from the Eurosystem (
Ý?
�
,
௧
â??
>
0
)
and
Φ
is the
standardized normal
cumulative distribution function.
In the selected sample wi
th
Ý?
�
,
௧
=
1
:
ܧ
[
Ý?
�
,
௧
|
Ý?
�
,
௧
=
1
]
=
ß?
â?²
Ý?
�
,
௧
+
�
�
�
�
(
ß?
â?²
Ý?
�
,
௧
)
where
�
is the inverse
Mills ratio
.
33
The model is estimated by maximum likelihood, which provides
(
Greene
, 2018).
Th
e selection equation includes
additional variable
s that are
not in the regression
model, namely
LCR,
and
variation of sight deposits
.
As before
, we run the model over the entire
sample period and over
the
COVID
–
19 period.
The results are
reported
in Table
10
A
.
The findings
are broadly consistent with the hypothesi
s that
exists a connection between
bank
s
â??
ability to
realize high value of LTR
and their lending
to the real economy.
4
.2
The collateral relaxation measures
In this subsection we
analys
e
the role of collateral eligibility for the transmission of central bank
liquidity provisions in the period following the outbreak of the COVID
–
19.
In order to participate to
the expansionary injections of liquidity proposed by the ECB, banks needed to hav
e
sufficient
availability of eligible
collateral
.
T
his constraint is relaxed if
new
become
eligible to be pledged
at the central bank
or
collateral valuation haircuts
are lowered
.
As described before, Eurosystem
introduced
relevant
measures
that effectively
eas
ed the
collateral
requirements.
We evaluate the impact of these measures on the amount of credit granted to non
–
financial sector.
First, we
investigate
if banks showing
high LTR
s
before the pandemic have been more inclined
to
grant loans to the real economy
than others.
We consider the level of LTR as of February 2020.
The
intuition is that banks with
high LTR
s
might
have
had a greater propensity to exploit
the
collateral
relaxation
measures decided by the Eurosystem
,
compared
to
banks with
low LTRs
, because they
have
probably
already pledged a large portion of their non
–
marketable assets
in the collateral pool.
Thus, these banks should have been the main beneficiaries of the new measures.
In the estimates, we
consider
a dummy
variable equal to 1 if
LTR of a bank
i
is greater than
0.
40 as of February 2020.
33
The inverse Mills ratio is the ratio of the probability density function to the complementary cumul
ative distribution
function
(the latter
also called survival function
).
26
The value of 0.40
represents the median of the historical
distribution.
34
Our choice is
to use banksâ??
LTR
as a source of heterogeneity
and to
focus
on those
one
that
actively
policy. For this reason we interact the LTR variable with a
second
dummy that takes value
equal to
1
for banks that have
a positive Eurosystem refinancing uptake in the period following the outbreak of
COVID
–
19 and zero
otherwise.
Indeed,
after the announcement of collateral easing measures,
lending patterns
of TLTRO
–
III
participating banks with a high value of LTR markedly
diverged
from those of
other banks. The
former banks experienced a sharp increase in outstanding total credits (see Figure
4
A).
We estimate the fol
lowing triple
–
interaction model:
Ü®Ý?Ý?
Ý?Ý?ܽÝ?Ý
�
,
௧
=
â?
�
+
ß?
1
�
(
ܮܴܶ
�
)
Ã?
�
�����
â??
���
à³?
Ã?
�
஼���
���஺�஺����
+
ß?
2
ܥܧܶ
1
ܴܽÝ�Ý?
�
,
௧
â??
1
+
�
�
,
௧
The dependent variable is the log
outstanding amount
of
credit granted
by bank
i
at time
t
.
As
described before, the variable
�
(
ܮܴܶ
�
)
is a dummy variable;
�
�����
â??
���
is a dummy equal to one for
banks that have
a positive Eurosystem refinancing uptake in the period following the outbreak of
COVID
–
1
9
and equal to zero for the non
–
participating bank
s
.
�
஼���
���஺�஺����
i
s a dummy equal to
1
in the period following the introduction of collateral relaxation measures by the Eurosystem (
t
�
April
20
20). We include the
atio
as
control variable
for
the
quality of ba
nks and
bank fixed
effects
to absorb any bank time
–
invariant characteristic
.
We show t
he estimation results in Table
11
A
.
The estimated triple interaction term suggests that
banks
with high LTR
have contributed
to the growth of credit supply
more than the other banks in
the period following the Eurosystemâ??s collateral measures.
35
These findings are consistent with
the idea that
in the COVID
–
19 period Eurosystem,
by injecting
liquidity
against a wid
er range of eligible collateral,
has
support
ed the provision of credit to the real
economy
.
The collatera
l easing measures in response
to
the pandemic have been an essential
cornerstone to avoid a credit crunch, contributing to the growth of credit supply, especially
for
â??constrainedâ? banks (with high LTR or low LCR).
as collateral,
Euro
systemâ??s
measures ensure
d
that the b
anking sector would
have
retain
ed
access to
ample central bank liquidity at
favorable
term
s
(i.e. during
TLTRO
–
III).
T
he revised collateral
framework
has
thus
created
a supportive environment for banks to continue lendin
g to the real
economy.
34
As a robus
tness check, we also consider the
value
in correspondence
of the 60
th
percentile
. Results are reported in
Table 11A
.
35
Since we introduce
bank
and
time
fixed effects, the dummies
�
(
ܮܴܶ
�
)
and
�
�����
â??
���
à³?
and the double interaction
�
(
ܮܴܶ
�
)
Ã?
�
�����
â??
���
à³?
are dropped, because of collinearity.
In a second set of estimates, we use LCR as measure of
banksâ?? exposure to the collateral
easing interventions, considering the level of the variable as of February 2020. The
intuition is that banks with low level of LCR (which have scarce HQLA collateral, for a given level of net cash
outflows) might have benefited more from the collateral re
laxation decided by the Eurosystem compared to banks
with high LCR.
Th
e results, available on request, show
that banks with low LCR, which were more constrained before
the introduction of collateral relaxation measures, have contributed to the growth of cr
edit supply more than the other
banks.
27
5
Conclusions
This paper
focuses
on
the interaction between
the liquidity coverage ratio (
LCR
)
,
the composition of
the collateral pool
, bank characteristics
,
and lending activity. Counterparties can increase HQLA to
achieve their LCR requirement by pledging with the Eurosystem non
–
receiving central bank reserves in return.
The liquidity transformation rate
(LTR)
, introduced by
Har
tung (2020),
is a synthetic measure that
indicates how much HQLA a bank can obtain by
pledging
a
n
as collateral
with the Eurosystem
.
In this study, we have
analys
ed
how
bank characteristics correlate with
the
liquidity transformation
rate, using data at bank level. The econometric analysis, conducted on the Italian banking system in
the period October 2015
–
January 2022, shows the existence of statistically significant relationships
between the liquidity tran
sformation rate and banksâ?? liquidity and efficiency. In particular,
we find
a
concave non
–
linear r
elationship between LCR and LTR
, which
implies that banks with liquidity ratios
close to the regulatory threshold are not more â??aggressiveâ? than the other ban
ks in exploiting the
differences between the haircuts and the eligibility criteria of the Eurosystem collateral framework
and those laid down in the LCR regulation. As a
caveat
, it should be emphasized that in recent years
almost all banks h
ave recorded
LCR
s
much
higher than the regulatory threshold.
Furthermore, it
emerges
that banks with high ROA
and high IT expenses
(measure
s
of efficiency) used more
These results are broadly confirm
ed
al
so in the COVID
–
19 period.
I
f
we consider
the entire sample
w
e do not find any correlation with financial
soundness
indicators
,
but
we obtain mixed results over the COVID
–
period, where, in some specifications of the model, LTR
Overall
,
we
find
no evidence
of credit risk
transfer
from banks to the central
bank. This supports the
view that central banks can provide liquidity and relax the collateral framework without absorbing
undue risks onto their balance sheet
s
, even
in situations of market stress.
Offering this form of
liquidity transformation can
improve liquidity in financial markets support
ing
financial stability
as
well
.
In
t
his
paper
, we
ha
ve
also investigated how LTR
is related to
credit supply. We find that
banks that
pledge
d
increase
d
their lending to the
economy
the most
. There
are at leas
t four
reasons to explain this result. First,
a bank
pledg
ing
nonâ??marketable assets
in the collateral pool
is more insured against price variations, given that credit
claims are less
proâ??cyclical
,
since their valuation only depends on their default probability
(
Barthélémy
et al.
, 2017)
.
Second
,
accept
ing credit claims as collateral,
the central bank relax
es
the
borrowing constraint of banks
(Ahn
et al.
, 2016). Third,
when a bank receives
credit from the central
bank posti
ng non
–
This bank
may
become
more willing
to take actions
, such as
the provision of additional credit
to the real economy,
that bring
its LCR ba
ck to the starting value
(Gocheva
et al.
, 2022
).
Fourth
,
the liquidity transformation rate can
be considered
as
an indicator of banksâ??
efficiency in the
liquidity management
process
,
i.e.
minimizing the cost of liquidity as well as optimizing compliance
with regulatory constraints
:
e
fficient banks are more inclined to provide credit (Albanese and Ciocchetta, 2021).
28
F
inally
, we have
analys
ed the role of collateral
easing
measures, enacted by the ECB
in the period
following the outbreak of the COVID
–
19
,
in
the transmission of central bank liquidity provisions.
Our findings show that the collateral relaxation measures contributed to the growth of credit supply,
especially for â??constrainedâ? banks (with high LTR or low LCR). By temporarily expanding the set
of
assets eligible as collateral, Eurosystemâ??s measures ensured that the banking sector would
have
retain
ed
access to ample central bank liquidity at
favorable
terms, creating a supportive environment
for banks to continue lending to the real economy.
Recent
ly the Eurosystem has scaled back its
monetary policy
refinancing
, following
some
TLTROs
â??
repayment
s by the banks.
In the next future
,
e
xcess liquidity
in the financial system is
ex
pected to
decline
, driven by monetary policy tightening
.
Pandemic collateral easing measures introduced in
April 2020
are
gradually
going to phase
out
,
in three steps between July 2022 and March 2024
.
This
gradual phasing out allows ample time for the Eurosystemâ??s counterparties to adapt
.
In any case, the
s
e
dyna
mic
s
should not lead a change
in the
banksâ??
behavior
with reference to the use as collateral of
LTR
s
.
Banks that
incurred in relevant
expense
s
for
the
adoption of
IT
system
s
for
the pledging and monitoring of credit claims in the collateral pool
should continue to use loans as a
collate
.
In a context of reduction
of both the collateral pool and excess liquidity in the
financial system, banks could find convenient
to
remove
part of the
government bonds from the
and
to
use them as repo in the market,
continu
ing
to keep loans
in the pool
.
29
References
Ahn,
J.â??H., Bignon, V., Breton, R. and
A.
Martin (2016).
Interbank
market and central bank policy.
Federal Reserve Bank of New York, Staff Report 763.
Albanese,
A. and F. Ciocchetta
(2021).
W
hich banks were more effective in supporting credit supply
during the pandemic?
Banca dâ??Italia
,
COVID
–
19 Note.
Altavilla, C., Barbi
ero, F., Boucinha, M. and L. Burlon (2020).
The great lockdown: pandemic
response policies and bank lending conditi
ons. ECB Working Paper Series, 2465.
Andreeva,
D.
and M. Garcia
–
Posada (2019).
The impact of the ECBâ??s targeted long
–
term
refinancing
operations on banksâ?? lending po
Banco de Espana Working
Paper No.
1903.
Barthélémy
,
J.
, V
.
Bignon
and B.
Nguyen
(2017).
Monetary policy, illiquid collateral and bank
lending during the European sovereign debt crisis
.
Economics and
S
tatistics n° 494
–
495
–
496.
Branzoli, N.,
E.
Rainone and
I. Supino (2021).
The Role of Banks’ Technology Adoption in Credit
Markets during the Pandemic
.
Available at SSRN:
https://s
srn.com/abstract=3878254
or
http://dx.doi.org/10.2139/ssrn.3878254
.
Carpinelli, L. and M.
Crosignani
(2020).
The Design and Transmission of Central Bank Liquidity
Provisions
.
Journal of Financial Economics
.
C
hamberlain, G.
(1984).
Panel Data,
Volume 2, ed. Z.
Griliches and
M.D. Intriligator. Amsterdam: North Holland, 1248
–
1318.
Chan, C., A. Duquerroy and W. Mullins
(2018)
.
lending
relationships.
Banque de France Working Paper No. 659.
Choi, D.
B.,
J. Santos
and T.
Yorulmazer
(2021).
A Theory of Collateral for the Lender of L
ast Resort
.
Review of Finance 25 (4), 973
–
996
.
de Guindos, L.
and I. Schnabel (2020).
Improving funding conditions for the real economy during
the COVID
–
19 crisis: the E
CBâ??s collateral easing measures. Blog E
CB
22/04/2020.
De Roure, C.
and
N. McLaren (2020).
Liquidity
and counterpar
ties
.
Bank of England
Staff Working Paper No. 830
.
ECB (2020a).
The euro area bank lending
survey
â??
First quarter of 2020
.
ECB (2020b).
The euro area bank lending
survey
â??
Second quarter of 2020
.
ECB (2020c).
The euro area bank lendin
g survey
â??
Third
quarter of 2020
.
ECB
Banking Supervision
(2020).
ECB Banking Supervision provides temporary capital and
operational relief
in reaction to coronavirus.
Press release,
12 March 2020.
Enria
, A. (2020).
Flexibility in supervision: how ECB Banking Supervision
is contributing to fighting
the econom
ic fallout from the coronavirus. Blog
27 March 2020.
Esposito, L., D. Fantino and Y.
Sung
(2020).
The impact of TLTRO2 on the Italian credit market:
.
Banca dâ??Italia Working Paper
No.
1264.
30
Fecht,
F., Nyborg, K. G., Rocholl, J.
and
J.
Woschitz (2016).
Collateral, Central Bank
Repos, and
Systemic Arbitrage.
Swiss Finance Institute Research Paper No. 16
–
66.
Gocheva
,
V
., Y.
Mudde
and J.
Tapking
(2022
).
Liquidity Coverage Ratios and Monetary
Policy
Credit in the times of Corona
.
ECB Working Paper Series, 2668
.
Grandia, R., P. Haenling, M.
Lo Russo
and P. Aberg
(2019)
, Availability of high
–
euro area. ECB Occasional Paper
Series, 218.
Greene, W.
H.
(
th edition.
Prentice Hall, US.
Hartung, B. (2020).
Liquidity transformation and Eurosystem credit
operations
.
ECB Mimeo.
Kedan,
D. and
A
.
Ventula Veghazy
(2021).
The implications of liquidity
policy implementation and the central bank balance sheet size: an empirical analysis of the euro area
.
ECB Working Paper
Series, (2515
).
K
iyotaki, N. and
J. Moore
(1997). Credit cycles.
Journal of
Political Economy, 105(2), 211
–
248.
Lane, P.R.
(2020).
The monetary policy res
ponse to the pandemic emergency. Blog E
CB
01/05/2020
.
Mundlak, Y.
(1978).
On the Pooling of Time Series and Cross Section
Data.
–
85.
Nyborg
, K.
G.
(
2015
).
Cent
ral Bank Collateral Frameworks.
Working paper, University
of Zurich and
Swiss Finance Institute.
Nyborg, K.
G.
(2016)
.
Collateral frameworks: The op
en secret of central banks.
Cambridge
University
Press, Cambridge, UK
.
Schmidt, K. (
2019
).
Does liquidity regulation impede the liquidity pr
o
fi
le of collateral?
ECB Working
Paper Series, 2256
.
Wooldridge, J.M.
(2013).
Correlated Random Effects Panel Data Models
.
I
nvited lecture,
IZA
Summer School in Labor Economics
.
31
Tables and figures
Figure
1
A
â??
Net outflows
and time
–
trend of
LCR
(a)
Outflows
(monthly data, total volume
)
(b)
LCR
(
monthly data
, percentage
)
Source: supervisory reports
,
harmonized at European level (consolidated for groups, individual for banks not belonging
to a group).
Figure
2
A
â??
L
iqu
idity buffer,
composition and trend
(a)
Composition
(monthly data, total volumes
)
(b)
Weights of components
(monthly data, percentage
)
Source: supervisory reports
,
harmonized at European level (consolidated for groups, individual for banks not
belonging to a group).
32
Figure
3
A
â??
Monetary
p
olicy
c
ollateral
p
ool,
time
trend and composition
(monthly data, value after haircut)
Source: Eurosystem
and Bank of Italy.
Figure
4
A
â??
Loans to non
–
financial sector of banks with high LTR
(monthly data; indices: 31 October 2015=100)
Source:
Eurosystem and
Bank of Italy.
(*) B
anks
with LTR�0.40
as of February 2020
and with
a positive Eurosystem
refinancing uptake in the period following the outbreak
of COVID
–
19 pandemic
.
33
Table
1
A
â??
Descriptive Statistics
Mean
Standard
Deviation
Min
Max
LTR
0.4143
0.3795
–
0.1299
1.0000
size
1.7753
0.9244
1.0000
4.0000
ROA
0.0017
0.0051
–
0.0012
0.0084
IT expenses
0.0020
0.0026
0.0000
0.0247
LCR
2
.
6947
1
.
6478
0.
5436
6
.
1383
0.1102
0.0839
0.0000
0.5555
AE
0.2436
0.1490
0.0000
0.8480
AEE
0.5255
0.2683
0.0000
1.0000
Sight deposits change
0.0122
0.0593
–
0.3126
3.1581
Strength
–
0.1359
4.0627
–
18.2335
2.7016
0.0211
0.0599
–
0.1388
0.4782
CET1 Ratio
0.1704
0.1155
–
0.0709
1.2414
NPL Ratio
0.1131
0.0904
0.0000
0.8540
Opportunity cost
0.0140
0.0050
0.0075
0.0290
0.1900
0.0342
0.1319
0.2314
34
Table
2A
â??
Correlation Matrix
LTR
size
ROA
IT
expenses
LCR
Refinancing
/ Total asset
AE
AEE
Sight
deposits
change
Strength
index bond
market
Net debt
position on
repo / Total
assets
CET1
Ratio
NPL Ratio
Opportunity
cost
Monetary
policy
haircut
(average)
LTR
1
size
0.3379
1
ROA
–
0.0873
0.0161
1
IT expenses
–
0.0159
–
0.0813
–
0.0376
1
LCR
–
0.18
–
0.2637
0.1219
0.1702
1
Refinancing / Total asset
–
0.1026
–
0.1299
0.0852
–
0.2407
0.0323
1
AE
0.3155
0.1569
–
0.067
–
0.2216
–
0.0713
0.4283
1
AEE
0.159
0.1393
–
0.0869
–
0.1509
–
0.0906
0.3912
0.7029
1
Sight deposits change
–
0.0271
–
0.0302
0.0174
–
0.0017
0.0152
0.0006
0.0059
–
0.0418
1
Strength
index bond market
0.0401
–
0.0083
0.063
0.0120
0.0212
0.0225
0.0192
–
0.0185
0.0023
1
Net debt position on repo / Total assets
0.1156
0.0851
0.1671
–
0.0703
–
0.1153
–
0.1399
0.1648
0.0584
0.0077
–
0.0276
1
CET1 Ratio
–
0.1512
–
0.1728
0.0203
0.0655
0.2633
0.1943
0.0789
–
0.0083
0.0183
–
0.0013
–
0.1058
1
NPL Ratio
–
0.0465
–
0.0783
–
0.281
0.0262
–
0.0087
–
0.073
–
0.1066
0.0932
0.0172
–
0.0482
–
0.1164
–
0.1089
1
Opportunity cost
0.0118
0.0132
–
0.0156
0.0069
0.0356
0.0726
0.0555
0.0031
–
0.0098
–
0.0083
–
0.0037
–
0.0021
–
0.1024
1
Monetary policy haircut (average)
–
0.0382
–
0.0232
–
0.0471
0.0139
–
0.1236
–
0.2103
–
0.0692
–
0.052
–
0.0204
–
0.0429
0.0589
–
0.0441
0.1435
–
0.1795
1
35
Table
3
A
â??
Panel regressions on LTR
Dep.
Variable:
LTR
(1)
(2)
(3)
â??Model:
Linear Panel
Linear
Panel
Tobit
Panel with
Mundlak
â??
Chamberlain
correction
L. size
0.0843
0.0628
0.0705***
(0.0599)
(0.0563)
(0.0133)
L. ROA
1.5179
1.4782
1.4967**
(2.8318)
(2.9252)
(0.7382)
L. IT expenses
7.3904
6.9227
7.7575***
(7.1387)
(6.6769)
(2.3946)
L.LCR
0.0129*
0.0111*
0.0101***
(0.0066)
(0.0064)
(0.0024)
L. Refinancing / Total Asset
–
0.6137***
–
0.6438***
–
0.7702***
(0.2087)
(0.2021)
(0.0607)
L.AE
0.6233***
0.5670***
0.6286***
(0.1689)
(0.1665)
(0.0403)
L.AEE
–
0.2528***
–
0.2384***
–
0.2564***
(0.0769)
(0.0747)
(0.0192)
L.
Sight deposits
change
–
0.0386
–
0.0424
–
0.0045
(0.0437)
(0.0451)
(0.0565)
L.
Strength
index bond market
–
0.0006***
–
0.0006***
–
0.0006
(0.0001)
(0.0001)
(0.0006)
L.
Net debt repo position /
Total Asset
0.0918
0.2061
0.0848
(0.2490)
(0.2375)
(0.0825)
L.
–
0.0097
–
0.0192
0.0015
(0.0760)
(0.0757)
(0.0474)
L.NPL Ratio
–
0.0980
–
0.0139
–
0.0219
(0.2313)
(0.2476)
(0.0464)
L.Opportunity cost
0.5529
2.2700
(1.0390)
(8.9388)
L.
Average
haircut
–
0.6616**
–
2.7448
–
3.2877***
(0.2612)
(2.2876)
(0.8321)
Bank Fixed effects
Yes
Yes
No
Time Fixed effects
No
Yes
Yes
Number of banks
87
87
8
7
Observations
5,836
5,836
5,836
Overall variance
0.1747
0
.1739
0.1801
Panel level variance
0.3238
0
.326
9
0.303
0
Bayesian Information
Criterion
–
3855.
047
–
3354.
395
–
1264.
292
Standard errors in parentheses: *** p0.01, ** p0.05, * p0.1.
L
before variables denotes that they are
lagged of order 1 (Lag 1).
36
Table 4
A
â??
Panel regressions on LTR
with a subset of variables
Dep.
Variable:
LTR
(1)
(2)
(3)
(4)
â??Model:
Linear
Panel
Linear
Panel
Tobit
Panel with
Mundlak
â??
Chamberlain
correction
Tobit
Panel with
Mundlak
â??
Chamberlain
correction
L. ROA
1.8034**
1.8186**
(0.7467)
(0.7455)
L. IT expenses
7.7805***
7.8798***
(2.4099)
(2.4077)
L.LCR
0.0542**
0.0407*
0.0280***
0.0273***
(0.0261)
(0.0234)
(0.0092)
(0.0092)
L.LCR
2
–
0.0054*
–
0.0037
–
0.0025*
–
0.0024*
(0.0032)
(0.0028)
(0.0013)
(0.0013)
L. Refinancing / Total Asset
–
0.7235**
–
0.8189***
–
1.0110***
–
1.0092***
(0.2768)
(0.2795)
(0.0841)
(0.0840)
L. Refinancing / Total Asset
×
Dummy banksmedian
0.0156
0.1217
0.2923***
0.2905***
(0.3076)
(0.3112)
(0.0883)
(0.0883)
L.AE
0.2795***
0.2510***
0.2845***
0.2840***
(0.0940)
(0.0907)
(0.0308)
(0.0308)
L.
Strength
index bond market
–
0.0003***
–
0.0004***
(0.0001)
(0.0001)
L.
Ratio
–
0.0752
–
0.0746
–
0.0275
(0.0778)
(0.0782)
(0.0478)
L.
NPL Ratio
–
0.1588
–
0.0971
–
0.1079**
–
0.1079**
(0.2298)
(0.2421)
(0.0469)
(0.0467)
L. Average haircut
–
0.5651**
–
1.5347
–
1.5019*
–
1.4907*
(0.2521)
(1.2099)
(0.8800)
(0.8800)
Dummy banksmedian
–
0.1388**
–
0.1343**
–
0.1626***
–
0.1633***
(0.0588)
(0.0619)
(0.0174)
(0.0174)
Bank Fixed effects
Yes
Yes
No
No
Time Fixed effects
No
Yes
Yes
Yes
Number of banks
89
89
8
7
8
7
Observations
5,986
5,986
5,
84
6
5,
84
6
Overall variance
0
.1770
0.1764
0.18
17
0.18
18
Panel level variance
0.3285
0.3315
0.3
361
0.33
77
Bayesian Information
Criterion
–
3753.26
–
3238.5
3
–
1200.79
–
1217.95
Standard errors in parentheses: *** p0.01, ** p0.05, * p0.1.
L
before variables denotes that they are lagged of order 1 (Lag 1).
37
Table 5
A
â??
COVID
–
19 period: panel regressions on LTR
Dep.
Variable:
LTR
(1)
(2)
(3)
(4)
â??Model:
Linear Panel
Linear
Panel
Tobit
Panel with
Mundlak
â??
Chamberlain
correction
Tobit
Panel with
Mundlak
â??
Chamberlain
correction
L. ROA
8.0912***
8
.
0484
***
(1.3376)
(1.3331
)
L. IT expenses
26.3973***
26
.
4611
***
(4.5326)
(4.5299
)
L.LCR
0.1316**
0.1160**
0.1093***
0.109
0***
(0.0543)
(0.0527)
(0.0178)
(0.0178)
L.LCR
2
–
0.0168**
–
0.0144**
–
0.0134***
–
0.0134
***
(0.0075)
(0.0072)
(0.0023)
(0.0023)
L. Refinancing / Total Asset
–
0.6968***
–
0.9519***
–
0.9295***
–
0.9261
***
(0.1801)
(0.2180)
(0.1245)
(0.1244
)
L. Refinancing / Total Asset
×
Dummy banksmedian
0.3391
0.0570
0.0032
0.0047
(0.2234)
(0.2576)
(0.1262)
(0.1257)
L.AE
0.2676*
0.2824*
0.2820***
0.2837
***
(0.1573)
(0.1677)
(0.0562)
(0.0559)
L.
Strength
index bond market
0.1028*
0.0971*
(0.0548)
(0.0545)
L.
Ratio
0.1039
–
0.0856
–
0.0554
(0.2256)
(0.2301)
(0.1489)
L.
NPL Ratio
0.2730
0.4181
0.4657***
0.4692
***
(0.4018)
(0.4434)
(0.0977)
(0.0974
)
L. Average haircut
–
0.8923***
–
1.5068***
–
1.3558***
–
1.3493
***
(0.2062)
(0.3431)
(0.2717)
(0.271
1)
Dummy banksmedian
–
0.0705*
–
0.0510
–
0.0678***
–
0.0676
***
(0.0419)
(0.0499)
(0.0190)
(0.0190)
Bank Fixed effects
Yes
Yes
No
No
Time Fixed effects
No
Yes
Yes
Yes
Number of banks
84
84
82
82
Observations
1,835
1,835
1,798
1,798
Overall variance
0.1123
0.110
8
0.1135
0.1135
Panel level variance
0.3397
0
.3415
0.2873
0
.2
885
Bayesian Information
Criterion
–
2836.655
–
2752.75
0
–
1715.
579
–
1729
.
746
Standard errors in parentheses: *** p0.01, ** p0.05, * p0.1.
L
before variables denotes that they are lagged of order 1 (Lag 1).
38
Table 6A
â??
Panel regressions on LTR
with focus on COVID
–
19 period
Dep. Variable:
LTR
(1)
(2)
(3)
(4)
â??Model:
Linear
Panel
Linear
Panel
Linear
Panel
Tobit
Panel with
Mundlak
â??
Chamberlain
correction
L. ROA
0.2299
(0.8257)
L. IT expenses
7.9207***
(2.4802)
L.LCR
0.0406*
0.0504**
0.0435*
0.0290***
(0.0232)
(0.0242)
(0.0234)
(0.0098)
L.LCR
2
–
0.0035
–
0.0052*
–
0.0043
–
0.0023
(0.0028)
(0.0029)
(0.0028)
(0.0014)
L. Refinancing / Total Asset
–
0.7272***
–
0.6969**
–
0.7667***
–
0.9831***
(0.2758)
(0.2735)
(0.2878)
(0.0852)
L. Refinancing / Total Asset
×
Dummy banksmedian
0.0445
0.0254
0.0998
0.2665***
(0.3039)
(0.3051)
(0.3125)
(0.0893)
L.AE
0.2783***
0.2774***
0.2579***
0.2896***
(0.0913)
(0.0914)
(0.0884)
(0.0307)
L.
Strength
index bond market
–
0.0002**
–
0.0002**
–
0.0003***
(0.0001)
(0.0001)
(0.0001)
L.
Ratio
–
0.0572
–
0.0527
–
0.0602
–
0.0352
(0.0816)
(0.0821)
(0.0836)
(0.0480)
L.
NPL
Ratio
–
0.1645
–
0.1963
–
0.1530
–
0.2545***
(0.2368)
(0.2351)
(0.2463)
(0.0531)
L. Average haircut
0.1228
–
0.0501
0.0895
1.7919
(0.4161)
(0.3917)
(1.6414)
(1.2952)
L.
ROA ×
Dummy
COVID19
5.7713***
(1.2779)
L. IT expenses ×
Dummy
COVID19
15.3788***
(2.7804)
L.
LCR
×
Dummy
COVID19
0.0357
0.0099
(0.0265)
(0.0203)
L.
LCR
2
×
Dummy
COVID19
–
0.0065*
–
0.0048*
(0.0038)
(0.0027)
L.
Dummy
COVID19
0.3148**
0.2849**
0.2173
0.3207***
(0.1397)
(0.1264)
(0.1331)
(0.0595)
L.
NPL ×
Dummy
COVID19
0.2465
0.2244
0.1769
0.3383***
(0.3372)
(0.3201)
(0.3405)
(0.0827)
Dummy banksmedian
–
0.1388**
–
0.1298**
–
0.1317**
–
0.1587***
(0.0588)
(0.0533)
(0.0578)
(0.0174)
Dummy COVID
–
19
–
0.0545
–
0.0284
(0.0336)
(0.0355)
Bank
Fixed effects
Yes
Yes
Yes
No
Time Fixed effects
No
No
Yes
Yes
Number of banks
89
89
89
8
7
Observations
5,986
5,986
5,986
5,845
Overall variance
0
.1761
0
.1763
0
.176
1
0.1803
Panel level variance
0
.3313
0
.3309
0
.332
3
0.3335
Bayesian Information
Criterion
–
3781.26
–
3780.46
–
3242.5
2
–
1251.
7
6
Standard errors in parentheses: *** p0.01, ** p0.05, * p0.1.
L
before variables denotes that they are lagged of order 1 (Lag 1).
39
Table 7A
â??
P
anel regressions on LTR
in the period February 2017
â??
February 2020
Dep.
Variable:
LTR
(1)
(2)
(3)
(4)
â??Model:
Linear
Panel
Linear
Panel
Tobit Panel with
Mundlak
â??
Chamberlain
correction
Tobit Panel with
Mundlak
â??
Chamberlain
correction
L. ROA
1.2614
1.232
8
(1.0097)
(1.0096
)
L. IT expenses
46.4794***
45.9754
***
(8.0746)
(8
.
01
3
9
)
L.LCR
0.0511*
0.0494*
0.0524***
0.0516
***
(0.0263)
(0.0249)
(0.0126)
(0.0125)
L.LCR
2
–
0.0052
–
0.0051
–
0.0054***
–
0.0054***
(0.0033)
(0.0032)
(0.0018)
(0.0018)
L. Refinancing /
Total Asset
–
0.2954
–
0.1787
–
0.7466***
–
0.7481
***
(0.4652)
(0.4946)
(0.2172)
(0.2164
)
L. Refinancing / Total Asset
×
Dummy banksmedian
0.0086
–
0.0234
0.4514**
0.4490**
(0.4736)
(0.4669)
(0.2107)
(0.2107)
L.AE
0.1554*
0.1271
0.2064***
0.2054
***
(0.0928)
(0.0900)
(0.0455)
(0.0455)
L.
Strength
index bond market
0.0002***
0.0001
(0.0000)
(0.0001)
L.
Ratio
0.0148
0.0288
–
0.0123
(0.0783)
(0.0780)
(0.0532)
L.
NPL Ratio
–
0.3560*
–
0.2979
–
0.2320***
–
0.2449
***
(0.2100)
(0.2348)
(0.0710)
(0.0702)
L. Average haircut
0.7020
–
3.0619
1.1370***
1.1074
***
(1.0451)
(4.9659)
(0.3870)
(0.3863
)
Dummy banksmedian
–
0.1217*
–
0.1157*
–
0.1216***
–
0.1219
***
(0.0633)
(0.0657)
(0.0340)
(0.0340
)
Bank Fixed effects
Yes
Yes
No
No
Time Fixed effects
No
Yes
Yes
Yes
Number of banks
87
87
85
85
Observations
2,795
2,795
2,725
2,726
Overall variance
0
.1454
0.1456
0.15
0
1
0.15
0
1
Panel level variance
0
.360
1
0
.3611
0.3
345
0.3
345
Bayesian Information
Criterion
–
2865.401
–
2631.365
–
1606.853
–
1
615
.
001
Standard errors in parentheses: *** p0.01, ** p0.05, * p0.1.
L
before variables denotes that they are lagged of order 1 (Lag 1).
40
Table
8
A
â??
Panel regressions on loans
Dep.
Variable:
Log loans
(1)
(2)
(3)
(4)
(5)
(6)
(7)
â??Model:
Linear
Panel
Linear
Panel
Linear
Panel
Linear
Panel
Linear
Panel
Linear
Panel
Linear
Panel
L.log(Loans)
0.9868***
(0.0063)
L.LTR
0.1411*
0.1930**
0.1754**
0.1756**
0.1755**
0.1751**
0.0061**
(0.0739)
(0.0845)
(0.0688)
(0.0686)
(0.0688)
(0.0687)
(0.0024)
L.log(Refinancing)
0.0729
0.0852
0.0856
0.0853
0.0863
–
0.0014
(0.0542)
(0.0515)
(0.0524)
(0.0515)
(0.0524)
(0.0020)
L.NPL Ratio
0.7309
0.7343
0.7312
0.7345
–
0.0028
(0.6044)
(0.6040)
(0.6046)
(0.6046)
(0.0223)
Ratio
0.1019
0.1040
0.1018
0.1042
–
0.0024
(0.2305)
(0.2308)
(0.2304)
(0.2312)
(0.0068)
â??
log(
GDP
)
380.387
***
–
5.5835
(138.0566)
(12.2189)
â??
Industrial production
0.1569***
(0.0529)
â??
business conf
idence
0.3972***
(0.1446)
L.
LTR
×
â??log(
GDP)
1.2643
0.0242
(0.7716)
(0.0558)
L.
LTR ×
â??
Industrial prod.
0.0014**
(0.0007)
L.
LTR
×
â??
business conf.
0.0004
(0.0005)
Bank Fixed effects
Yes
Yes
Yes
Yes
Yes
Yes
Yes
Time Fixed
effects
Yes
Yes
Yes
Yes
Yes
Yes
Yes
Number of ba
nks
87
83
83
83
83
83
83
Observations
5,973
5,350
5,284
5,215
5,215
5,215
5,215
R
–
squared
0.1543
0.2800
0.3021
0.3025
0.3023
0.3016
0.9763
Standard errors in parentheses: *** p0.01, ** p0.05, * p0.1.
L
before
variables denotes that they are
lagged of order
1 (Lag 1).
41
Table 9
A
â??
Panel regressions on loans
(mon�th February 2020)
Dep.
Variable:
Log loans
(1)
(2)
(3)
(4)
(5)
(6)
(7)
â??Model:
Linear
Panel
Linear
Panel
Linear
Panel
Linear
Panel
Linear
Panel
Linear
Panel
Linear
Panel
L.log(Loans)
0.9193***
(0.0186)
L.LTR
0.1013
0.1372*
0.1447**
0.1466**
0.1460**
0.1447**
0.0045
(0.0789)
(0.0749)
(0.0701)
(0.0698)
(0.0700)
(0.0702)
(0.0077)
L.log(Refinancing)
0.1088**
0.1101**
0.1090**
0.1104**
0.1102**
0.0019
(0.0542)
(0.0542)
(0.0536)
(0.0540)
(0.0541)
(0.0041)
L.NPL Ratio
–
0.1794
–
0.1617
–
0.1753
–
0.1803
–
0.0360
(0.4414)
(0.4514)
(0.4426)
(0.4454)
(0.0296)
–
0.8244
–
0.8410
–
0.8284
–
0.8244
0.0865*
(0.6038)
(0.6044)
(0.6034)
(0.6040)
(0.0508)
â??log(
GDP)
4.1494**
0.4677
(1.9398)
(0.3285)
â??
Industrial production
0.0187**
(0.0085)
â??
business confidence
0.2880**
(0.1280)
L.LTR ×
â??log(
GDP)
0.3769
0.0081
(0.4237)
(0.0626)
L.
LTR ×
â??
Industrial prod.
0.0010
(0.0006)
L.
LTR
×
â??
business conf.
–
0.0001
(0.0002)
Bank Fixed effects
Yes
Yes
Yes
Yes
Yes
Yes
Yes
Time Fixed effects
Yes
Yes
Yes
Yes
Yes
Yes
Yes
Number of ba
nks
83
80
80
80
80
80
80
Observations
1,766
1,679
1,676
1,676
1,676
1,676
1,676
R
–
squared
0.1412
0.3217
0.3303
0.3311
0.3312
0.3303
0.9038
Standard errors in parentheses: *** p0.01, ** p0.05, * p0.1.
L
before
variables denotes that they are
lagged of order 1 (Lag 1).
42
Table
10
A
â??
Heckman selection model on loans
(1)
Entire
period
(2)
Month �
feb. 2020
Dep. Variable for
Regression Equation:
Log loans
L.LTR
2.0
129
***
1.
7004
**
*
(0.50
33
)
(0.6
583
)
L.NPL Ratio
–
1.
2918
–
8.6
507
*
(2.
1865
)
(4.
8527
)
–
1.0
230
–
10.
2506
*
(1.2
49
9)
(
6
.
0026
)
Dep. Variable for
Selection Equation:
Positive liquidity uptake
L.LTR
0.1
302
0.24
15
(0.1614)
(0.1
693
)
L.NPL Ratio
0.0628
–
1.1567
(0.7419)
(0.8906)
0.6222
–
0.5304
(
0.4578
)
(
0.6046
)
L.LCR
–
0.0004
0.0062
(
0.0045
)
(
0.0056
)
L.Sight deposits change
0.3695
–
0.8142*
(
0.4490
)
(
0.4873
)
Ath.rho
–
0.2825
1.6580***
(
1.0189
)
(
0.4134
)
Ln.sigma
0.5719***
1.0027***
(
0.1913
)
(
0.1370
)
Number of banks
89
84
Observations
5,805
1,757
Log
pseudolikelihood
–
4728.991
–
1452.176
Standard errors in parentheses: *** p0.01, ** p0.05, *
p0.1.
L
before
variables denotes that they are
lagged of order
1 (Lag 1).
Ath.rho
estimates the inverse
hyperbolic tangent of
correlation between the regression equation residual and the
selection equation residual, Ln.sigma estimates the logarithm
of standard error of the residual in the regression equation.
43
Table
11
A
â??
Panel regressions on
loans
with dummy LTR
Dep.
Variable:
Log loans
(1)
(2)
(3)
(4)
(5)
(6)
(7
)
(
8
)
â??Model:
Linear
Panel
Linear
Panel
Linear
Panel
Linear
Panel
Linear
Panel
Linear
Panel
Linear
Panel
Linear
Panel
dummy_collateral_relaxation
×
dummy_ECB_uptake�0
×
dummy_LTR�50
th
perc.
0.3046*
0.2582*
0.2355
0.2835*
(0.1556)
(0.1419)
(0.1489
)
(0.1619)
dummy_collateral_relaxation
×
dummy_ECB_uptake�0
×
dummy_LTR�60
th
perc.
0.3250**
0.2770*
0.2547*
0.3042*
(0.1560)
(0.1422)
(0.1491)
(0.1622)
–
0.4215
–
0.4253
–
0.4253
–
0.4292
(0.2843)
(0.2827)
(0.2638)
(0.2623)
Bank Fixed effects
Yes
Yes
Yes
Yes
Yes
Yes
Yes
Yes
Time Fixed effects
No
No
No
No
Yes
Yes
Yes
Yes
Number of banks
87
87
87
87
87
87
87
87
Observations
6,053
6,053
5,973
5,973
5,973
5,973
6,053
6,053
Overall variance
0.246
2
0.2463
0.243
5
0.2436
0.2393
0.2394
0.
2421
0.
2422
Panel level variance
1.991
1
1.990
3
1.983
6
1.982
8
1.9830
1.9821
1.
9908
1.9
900
R
–
squared
0.1253
0.1243
0.1318
0.1309
0.1718
0.1710
0.
1647
0.
1637
Standard errors in parentheses: *** p0.01, ** p0.05, * p0.1.
L
before variables denotes that they are lagged of order 1 (Lag 1).
n. 1
Marino, Luca Mibelli, Laura Ricciardi and Giovanni M. Sabelli
ONAL
n. 2
by Mauro Arcese, Domenico Di Giulio and Vitangelo Lasorella
n. 3
Green Bonds: the Sovereign Issuers’ Perspective,
by Raffaele Doronzo, Vittorio Siracusa and
n. 4
central bank money,
by Cristina Mastropasqua, Alessandro Intonti, Michael Jennings, Clara
Mandolini, Massimo Maniero, Stefano Vespucci and Diego Toma
ONAL
n. 5
analysis with Bitcoin and other infrastructures,
n. 6
Proposal for a common categorisation of IT incidents,
Bundesbank, European Central Bank, Federal Reserve Board, Financial Conduct Authority,
Ministero dell’Economia e delle Finanze, Prudential Regulation Authority, U.S. Treasury
ONAL
n. 7
Inside the black box: tools for understanding cash circulation,
by Luca Baldo, Elisa Bonifacio,
Marco Brandi, Michelina Lo Russo, Gianluca Maddaloni, Andrea Nobili, Giorgia Rocco,
Gabriele Sene and Massimo Valentini
n. 8
The impact of the pandemic on the use of payment instruments in Italy,
by Guerino Ardizzi,
Alessandro Gambini, Andrea Nobili, Emanuele Pimpini and Giorgia Rocco
P
n. 9
by Paolo Bramini,
Valentini
ONAL
n. 10
A digital euro: a contribution to the discussion on technical design choices,
by Emanuele Urbinati,
Alessia Belsito, Daniele Cani, Angela Caporrini, Marco Capotosto, Simone Folino, Giuseppe
NST
TUT
ONAL
I
SSUES
n. 11
From SMP to PEPP: a further look at the risk endogeneity of the Central Bank,
by Marco
n. 12
TLTROs and collateral availability in Italy,
by Annino Agnes, Paola Antilici and Gianluca
Overview of central banks’ in-house credit assessment systems in the euro area, by L
Auria, Markus Bingmer, Carlos Mateo Caicedo Graciano, Clémence Charavel, Sergio Gavilá,
Alessandra Iannamorelli, Aviram Levy, Alfredo Maldonado, Florian Resch, Anna Maria Rossi
ONAL
n. 14
The strategic allocation and sustainability of central banks’ investment, by
Davide Di Zio,
ESEA
CH
P
n. 15
Climate and environmental risks: measuring the exposure of investments, by
Ivan Faiella
Johnny Di Giampaolo
and Davide Nasti
ERS
UBLISHED
IN
THE
‘M
ARKETS
,
NFRASTRUCTURES
, P
AY
ENT
YSTE
’
SERIES
n. 16
Mechanism, by
Fabrizio Dinacci and Ann Börestam
n. 17
Daniela Della Gatta
ONAL
n. 18
Cyber resilience per la continuità di servizio del sistema nanziario, by
and Antonino Fazio
ONAL
n. 19
Francesco Di Stasio, Carlo Palmers, Faisal Alhijawi, Erol Kaya, Christophe Piccarelle, Stuart
Butler, Jwallant Vasani, Giancarlo Esposito, Alberto Tiberino and Manfredi Caracausi
n. 20
n. 21
NST
TUT
ONAL
I
SSUES
n. 22
by Paola Antilici, Gianluca Mosconi and Luigi Russo
ONAL
n. 23
by Guerino
Ardizzi and Massimiliano Cologgi
n. 24
Press news and social media in credit risk assessment: the experience of Banca d’Italia’s
house Credit Assessment System,
by Giulio Gariano and Gianluca Viggiano
The bonre of banknotes,
by Michele Manna
n. 26
Matteo Caruso, Stefano Cossu, Giuseppe Galano, Simone Mancini, Gabriele Marcelli, Piero
Martella, Matteo Nardelli and Ciro Oliviero
n. 27
by Guerino Ardizzi and Alessandra Righi
ONAL
I
n. 28
TIPS: a zero-downtime platform powered by automation, by
Gianluca Caricato, Marco
n. 29
Marc Glowka, Alexander Müller,
Livia Polo Friz, Sara Testi, Massimo Valentini and Stefano Vespucci
ONAL
n. 30
by Massimiliano
Cologgi
n. 31
Open Banking in the payment system: infrastructural evolution, innovation and security,
supervisory and oversight practices,
by Roberto Pellitteri, Ravenio Parrini, Carlo Cafarotti
ONAL