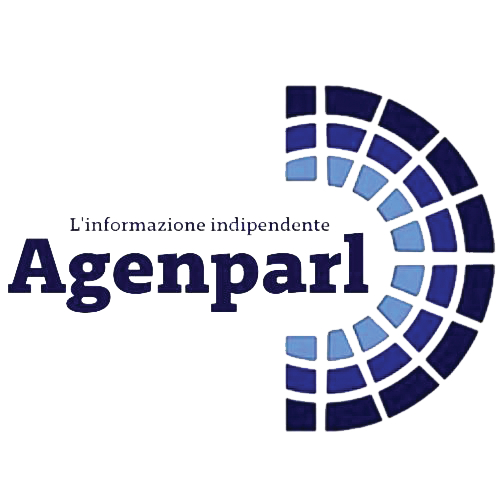
(AGENPARL) – STANFORD (CA) ven 17 marzo 2023
This table provides a compendium of AI core principles that I refer to simply as the “Core Principles.” Some of these were compiled from publications issued by OECD, UNESCO, IEEE, ISO, NIST, FTC, G20, and APEC. The AI Core Principles are applicable to the entire AI life cycle. This table is intended as a reference point that can be used by various stakeholders to develop common law (courts), regulations, drafting contracts, audit, design, etc.
The Core Principles are divided into three categories: Operational (Green), Value (Orange), and Both (Blue). Operational means the Core Principle is primarily related to the enabling the operational characteristics of the AI app. Value means it is primarily related to promoting values/norms. Both means the Core Principle combines operational and value elements.
This table will be periodically updated.
Core Principle | What it means and aims to promote | |
1 | Accessibility | Affordable; user friendly interface and experience (UI/UX) methods; facilitates end user understanding of the algorithm. |
2 | Accountability | Examines output (decision-making or prediction); identifies gaps between predicted and achieved outcomes. |
3 | Accuracy | Uses credible data (authentic, non-repudiated, protected from unauthorized modification or destruction); dataset is derived by following reasonable selection criteria to minimize harm; input and output measurement capable. |
4 | Bias | Protects against disparate impact, the increase of discrimination against protected classes, unjust outcome; more generally, protects against inaccurate results; a subset of ethics. |
5 | Big data | Uses high-quality data; compliant with decreasing dependence on labeled data architectures; maintains contextual relevance; promotes data accessibility. |
6 | Consent | Aligns with the end user’s consent to the application’s objectives. |
7 | Cooperation | Facilitates global development; facilitates internal and external information sharing which maps also to transparency. |
8 | Efficiency | Supports a cost-effective training to time ratio; makes optimal decisions with respect to achieving objective and resource utilization. |
9 | Enabling | Compliant with government sponsored controlled environments for testing and scaling AI. |
10 | Equity | Protects against widening gender and protected class gaps; maps to bias. |
11 | Ethics | Encompasses a broad range of values that aim to eliminate or reduce risk to human[x]life, privacy and property and enhance and maintain public trust. |
12 | Explainability (XAI) | Enables understanding of outcomes and operation; enhances accountability; enhances transparency. |
13 | Fairness | Supports treatment based on similar characteristics, policies, and procedures to manage against unintended disparate treatment; reduces unexpected outcomes; uses anonymized or pseudonymized data. |
14 | Fidelity | Supports measuring of the application’s performance relative to its code and across the deployment population; supports measure of ongoing compliance with the Core Principles. |
15 | Fundamental rights | Open data access compliant, in contrast to use of closed (proprietary) models that inhibit access; maps to accessibility. |
16 | Governance | Developed within an environment that follows documented policies and procedures that maintain consistent data quality. |
17 |
Human-centered
|
Compatible with law, privacy, human rights, democratic values, and diversity; contains safeguards to ensure a fair and just society; protects against augmenting and perpetuating social disparity, promotes equality, social justice, consumer rights; aligns with best practices in user interface and experience (UI/UX); human-collaborative compatible; compatible with experiential AI (human-in-the-loop); maps to human-like dexterity and adaptability in robotic applications. |
18 |
Inclusive
|
Widespread contribution to society; does not exclude certain parts of society; consistent with the Core Principle of ethics. |
19 | Interpretability | Interchangeable with explainability; maps to trust. |
20 | Metrics | Capable of measuring degree of compliance and effectiveness with the Core Principles; promotes standardization. |
21 | Permit | Application development and end user access are compliant with a permit. |
22 | Predictable | Maintains Core Principle compatibility throughout its lifecycle. |
23 | Privacy | Maintains data protection; compatible with data minimization principles and Fair Information Principles. Compatible with and maintains the state of anonymized, pseudonymized, and encrypted data; resistant to re-identification. |
24 | R&D | Promotes research; emphasizes keeping human at the center of AI development (also referred to as human-in-the-loop, see Experiential AI). |
25 | Reliability | Design, development, and deployment follow best practices and promote select Core Principles; deployment takes a lifecycle perspective and includes patching AI; maintains data credibility; follows compliance by design; does not materially deviate from coded objective; algorithmic recidivism is accounted for, monitored, and corrected. |
26 | Resilience | Failure recovery capable. |
27 | Robust | Operates with minimum downtime; resistant to adversarial attacks; maintains operational integrity throughout its lifecycle; able to identify and handle input/output unreliability; resistant to unintended behavior from the end user; exhibits high degree of problem flexibility; autonomous behavior maintains line of sight with human developer and end user; accommodates information sharing best practices; uses sophisticated learning techniques to minimize bias. |
28 | Safety | Minimizes unintended behavior; follows permit-related policies and procedures. |
29 | Security | Resistant to adversarial attacks and inference attacks; compatible with information sharing best practices. |
30 |
Sustainable
|
Promotes long-term growth capabilities; compatible with to information sharing best practices. |
31 | Track record | Application is the product of a developer known for designing AI compatible with the Core Principles. |
32 | Transparency | Promotes disclosure, discovery, accessibility, and non-discriminatory output; enables end-user understanding; promotes consensus; enables audit; compatible with experiential AI. |
33 | Trustworthy | A catchall for: accuracy, explainability, interpretability, privacy, reliability, robustness, safety, security (resilience), and bias. |
34 | Truth | Does not cause unfair or deceptive outcomes. |
35 | Wherewithal | Developer is financially sound, has operational resilience, and implements policies and procedures to fully support Core Principle compliant AI. |
36 | Workforce Compatible | Considerate of issues relative to worker displacement; promotes effective worker use, interaction, and training with AI. |
Fonte/Source: https://law.stanford.edu/2023/03/17/ai-life-cycle-core-principles/